COVID-19 Updates
COVID-19 Science Update for March 27th: Super-Spreaders and the Need for New Prediction Models
Absent isolation or other precautionary measures, the average socially active COVID-19 infectee will transmit the disease to an average of about 2.4 people. i.e., the R0 value is 2.4. But super-spreaders can spread a disease to dozens or hundreds.
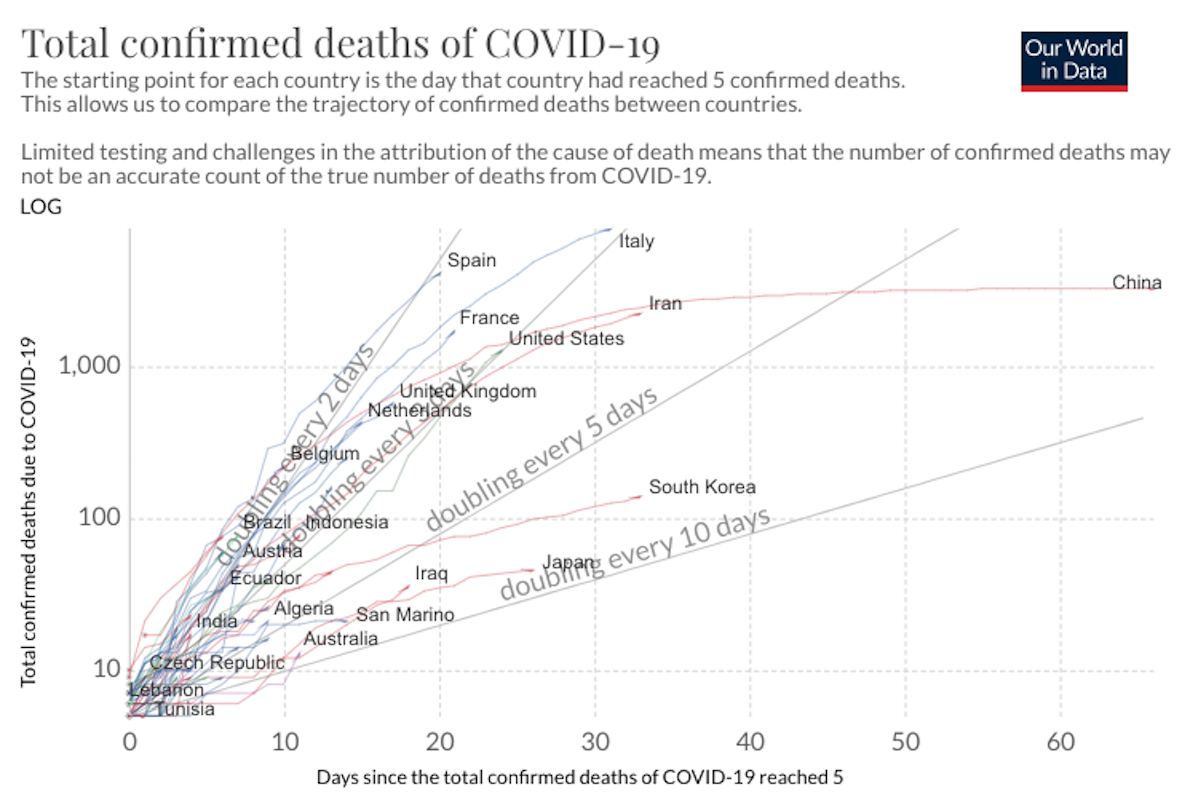
This article constitutes the March 27th, 2020 entry in the daily Quillette series COVID-19 UPDATES. Please report needed corrections or suggestions to [email protected].
According to statistics compiled by Our World in Data (OWD), the number of newly reported COVID-19 deaths increased yesterday. There were 2,681 new confirmed COVID-19 fatalities globally, compared to 2,423 reported on yesterday. This was largely due to increased death tallies in France (365 new deaths, up from 231 the day before), Italy (660, down from 685 reported on Thursday and 743 reported on Wednesday), Spain (655, down from 738), and the United States (246, virtually unchanged from Thursday’s report of 249, with the New York City area remaining the pandemic’s American epicenter).
On Wednesday, I mentioned that just four countries—France, Italy, Spain, and the United States—represented 78 percent of that day’s newly reported global COVID-19 deaths. In yesterday’s reports, it was 79 percent. In today’s reports, it is 72 percent. This figure has remained above 65 percent for 17 of the last 18 days. In these four countries, the annualized per-capita death rate from COVID-19 during this 18-day period has been 0.06 percent, or about one per 1,640. In the rest of the world, the annualized rate has been 0.00016 percent, or about one per 625,000.
I produced the chart above using OWD’s data-manipulation tools, which allow users to customize charts by nation and time period. One especially useful feature that OWD has been updating in recent days plots total confirmed COVID-19 deaths against time using a logarithmic scale on the vertical axis. This improves comprehensibility for those who have difficulty in conceptualizing exponential growth patterns. The chart serves to convert a constant rate of exponential growth into a straight line that can be plotted in a way that represents X-day doubling periods, as can be seen on the figure below. When the play button is pressed, the figure animate, and the trend lines progress chronologically from January 21st onward.
It may take readers a minute or two to grasp what the figure is showing. But it is worth the time, because there is no other data-presentation technique (that I know of) which captures the extent of COVID-19’s spread so vividly. (I had never used OWD’s resources before the COVID-19 crisis hit. But I now find the site indispensable. And I think many readers will find that their existing go-to media outlets are either adapting or reproducing OWD’s data and graphs.)
A low position on the vertical axis is obviously better than a high position—since this corresponds to fewer total deaths. But the slope of the line is even more important: A steep slope means a high rate of exponential growth in deaths, while a flat slope (China is almost there) means no new deaths at all. Even more important than that is the curvature of each line: A line that becomes less steep over time corresponds to a nation that is lowering its rate of exponential growth.
And here we get to the good news: With some exceptions, all depicted nations feature lines that are either straight or curving in the desired direction. The bad news is that the lines corresponding to the aforementioned four nations—France, Italy, Spain and the United States—already are generating hundreds of deaths every day, which means that even if their growth rates ebb in relative terms, the daily death toll will remain high for weeks to come.
My simplistic comparison of these four countries to the rest of the world is misleading in a number of ways, of course. Several countries with enormous death tolls—China, most notably—endured their greatest casualties before March began. The virus has not yet begun to ravage most nations in the southern hemisphere, where the toll could eventually be worse. And to a certain extent, such patterns are predictable epidemiological artifacts anyway, because the countries where a pandemic strikes first usually serve as cautionary tales for others. The horrific spread of COVID-19 in Italy, in particular, put the rest of the world on notice that this disease would not be confined to East Asia, and helped convince doubters (of which I was one in the early days) that COVID-19 couldn’t be treated as just another variation on the seasonal flu.
This cautionary-tale effect helps explain the pronounced clustering phenomenon I’ve been focusing on in recent days, since it naturally inflates the difference between death tallies in the nations where the first outbreaks occur and those that have time to prepare. Yet as the WHO has noted, this unusually pronounced clustering effect also takes place on lower levels of geographical scale—and began manifesting even before the risks associated with COVID-19 were fully appreciated—for reasons that don’t yet seem to be entirely understood.
In this update, I will focus on one contributing factor to this clustering phenomenon: the role of so-called super-spreaders. In recent days, the media has been full of stories about such COVID-19 super-spreaders, including in Italy, South Korea, Britain, and Boston. These stories sometimes are presented as tragic one-off case studies. Yet their statistical impact is enormous. As of March 12th, for instance, roughly 80 percent of Massachusetts’ COVID-19 cases could be traced to a single corporate meeting. In Italy, genetic analyses suggest that the country’s epidemic originated with just two people.
Absent isolation or other precautionary measures, the average socially active COVID-19 infectee will transmit the disease to an average of about 2.4 people. i.e., the R0 value is 2.4. But super-spreaders can spread a disease to dozens or hundreds. Studying the outsized role played by super-spreaders may not only go a long way toward explaining the clustering of COVID-19 cases, but also help policymakers optimize resource allocation in the fight to suppress COVID-19 or otherwise “flatten the curve” associated with its spread.
Super-spreading isn’t confined to COVID-19. Mary Mallon (an asymptomatic typhoid carrier) famously infected more than 50 Americans. One study of a tuberculosis ward found that three patients (out of a group of 77) accounted for almost three-quarters of new infections. As early as the 1960s, researchers discovered that so-called “cloud babies” (an incredibly creepy term) could spread Staphylococcus aureus around nurseries at extraordinary rates. And one reason that South Korea was so effective at suppressing the spread of COVID-19 is that the country already had dealt with a MERS super-spreading event (known in the literature as an “SSE”) in 2015, a precedent that offered important parallels to the challenge posed by COVID-19.
In a 2016 paper, South Korean doctor Byung Chul Chun noted that the MERS outbreak could be summarized as:
an explosive epidemic by infrequent super-spreaders. The number of secondary cases in the transmission tree was extremely skewed. Among 186 confirmed cases, 166 cases (89.2%) did not lead to any secondary cases, but 5 (2.7%) super-spreaders lead to 154 secondary cases. The imported index case [i.e. original case] was a super-spreader who transmitted the MERS virus to 28 people (referred to as secondary cases), and 3 of these secondary cases became super-spreaders who infected 84, 23 and 7 people, respectively. Eighty-four secondary cases resulting from a single case is one of the largest numbers observed in a SSE since the SARS outbreak in Prince of Wales Hospital in Hong Kong. None of the super-spreaders in the MERS outbreak in Korea was a healthcare worker.
I will return to Dr. Chun later in this article. But at this point, I’ll pivot back to COVID-19, and recommend an early-release version of a June, 2020 Centers for Disease Control and Prevention (CDC) report, Identifying and Interrupting Superspreading Events—Implications for Control of Severe Acute Respiratory Syndrome Coronavirus 2, by Thomas R. Frieden and Christopher T. Lee. Echoing points made by Dr. Chun and others, the authors note, “SSEs highlight a major limitation of the concept of R0,” since R0, being a mean or median value “does not capture the heterogeneity of transmission among infected persons.”
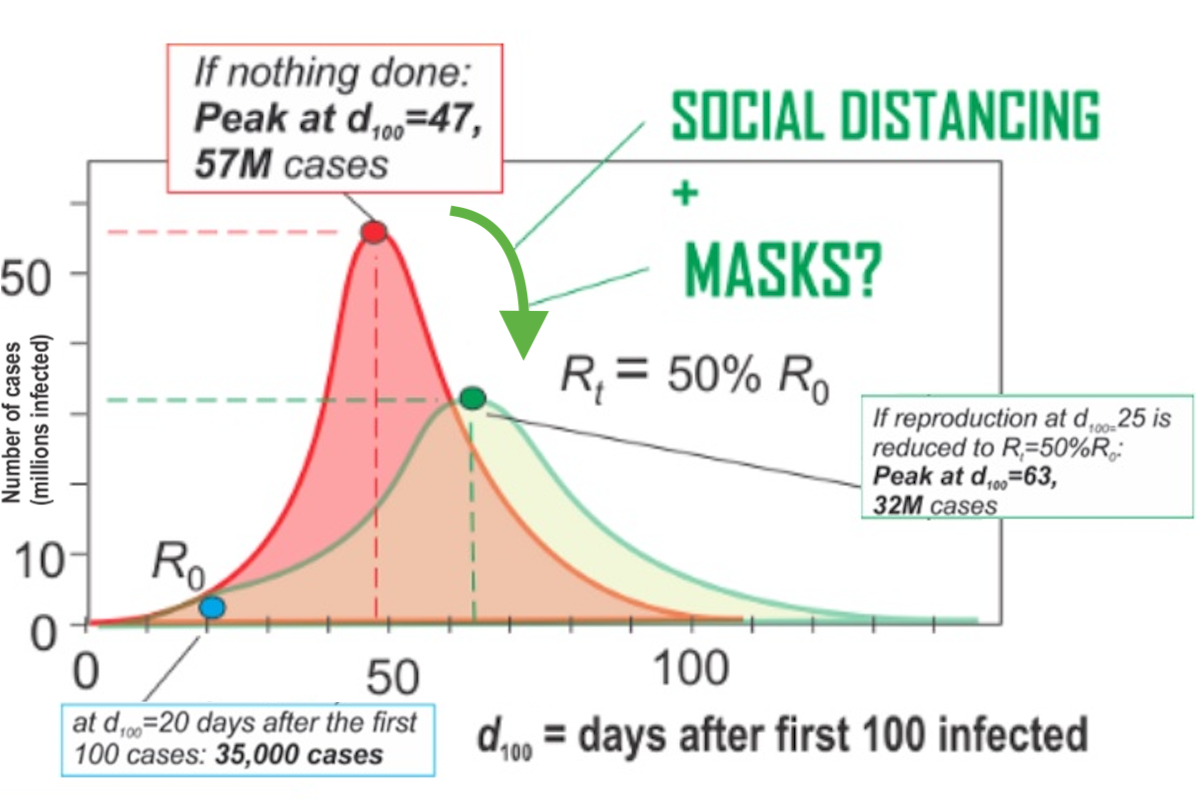
I wrote about the epidemiological concept of R0 in Wednesday’s update because R0 lies at the heart of all those COVID-19 projections we see in the media. At the most basic level of analysis, computer modelers apply an R0 figure to some baseline pool of infected individuals, and then iterate the spread of the disease exponentially over time. But as I noted, the idea of R0 is based on the premise that people behave with some constancy over time, since the value isn’t an inherent biological constant associated with any particular pathogen; it’s basically a composite statistic that imputes everything from human sociology to hygiene practices to environmental conditions. And it can change in an instant when people are told to, oh, say, avoid sneezing in each other’s faces. And since R0-based models are (like disease spread itself) non-linear systems typically based on large numbers of iterations, even small changes in effective R0 can lead to wildly divergent values. That’s why the same British expert who very recently warned us of 500,000 COVID-19 deaths in Britain now says he expects fewer than 20,000.
In fact, one of the long-term effects of the COVID-19 crisis might be to accelerate a shift toward models that are less rooted in traditional R0 frameworks. Thanks to smartphones, the velocity of public-health information is now so high, and the penetration of that information so thorough, that prescribed behavioral changes and direct public interventions can radically disrupt disease transmission dynamics many times over within the time scale of a single pathogenic incubation period. In Wuhan, according to unpublished CDC data, the observed R0 for COVID-19 went from 3.86 to 0.32 in just a few weeks. On the Diamond Princess cruise ship, the rate went from about 15 to less than two once isolation protocols commenced.
What differentiates SSE generators from other infected individuals? The CDC authors go through a lengthy laundry list of possible factors, including possible variations between multiple disease sub-types. But the unfortunate bottom line is that they don’t know. It is even theoretically possible that asymptomatic individuals—such as Typhoid Mary all those years ago—may generate SSEs. However, the CDC authors do note that there were no known examples of an SSE being traced to asymptomatic individuals during the SARS epidemic of 2002-2003 (this being the related virus strain SARS-CoV-1, as distinct from the SARS-CoV-2 pandemic we face now). So that’s good news.
Indeed, the whole issue of SSEs more generally seems somewhat mysterious, even to experts, in part because a systematic analysis of super-spreading behaviour is difficult unless you know how a person conducts himself throughout his personal and professional life—including how he coughs, talks, laughs, eats and conducts himself in the kitchen and bathroom. These are hard things to measure. Even the task of researching a single sneeze is difficult. “A physician colonized intranasally with S. aureus exhibited a 40-fold increased airborne dispersal after acquiring an upper respiratory rhinovirus infection, becoming thus a ‘cloud adult,’ ” wrote Richard A. Stein in a 2011 International Journal of Infectious Diseases article. “And a study that examined volunteers with S. aureus nasal carriage revealed, on average, a two-fold increase in bacterial dispersion into the air after rhinovirus infection, with up to 34-fold higher dispersion observed in one volunteer. This process is mechanistically insufficiently understood, and one scenario that was proposed is that rhinovirus-induced swelling of the nasal turbinates could create a high-speed airflow that establishes aerosols” (my emphasis, Dr. Stein’s euphemisms).
But even if we have no way of detecting super-spreaders beforehand, our emerging understanding of their massive contribution to the spread of epidemics should help drive the campaign for more COVID-19 testing—and faster testing. From Seattle to South Korea, many of the biggest outbreaks were fuelled by a small handful of very sick, highly symptomatic people who drifted along for days before their condition was correctly treated and isolated. (In South Korea, some have noted, the problem was exacerbated by patients who went “doctor shopping,” spreading their germs in many different clinics.) Test everyone who is symptomatic, and test them early, and you will prevent SSEs.
While we are at it, we need to stop wasting resources on pointless measures such as closing remote parks and natural reserves, where few people come close to one another anyway. In an especially important section of the aforementioned CDC report, the authors note that even COVID-19 super-spreaders can’t seem to infect people effectively in open spaces: “Rapid person-to-person transmission of COVID-19 appears likely to have occurred in healthcare settings, on a cruise ship, and in a church. In a study of 110 case-patients from 11 clusters in Japan, all clusters were associated with closed environments, including fitness centers, shared eating environments, and hospitals, [where] the odds for transmission from a primary case-patient were 18.7 times higher than in open-air environments.” These closed environments represent the sort of scenario we need to target—not British couples out on a jaunt to Sugar Loaf, Pen-y-Fan and other rustic destinations.
We also need to be increasingly wary of computer models that apply a traditional R0-based approach to a novel coronavirus amidst a real-time public-health mobilization campaign whose speed and scale are likely unprecedented in human history. Even long before COVID-19 was a thing, infectious-disease experts such as James Lloyd-Smith were arguing that “the distribution of individual infectiousness around R0 is often highly skewed”; that approaches accounting for super-spreaders do a better job modelling the sudden cluster-based boom-and-bust quality of many diseases; and, crucially for today’s policymakers, that such analyses show how, in these cases, “individual-specific control measures outperform population-wide measures.”
As part of his approach, Lloyd-Smith introduced the “individual reproductive number,” v, a “random variable representing the expected number of secondary cases caused by a particular infected individual… drawn from a continuous probability distribution with population mean R0 that encodes all variation in infectious histories of individuals, including properties of the host and pathogen and environmental circumstances.” In essence, what he’s doing here is atomizing R0 into a probability cloud and assigning each (theoretical version) of us our own personal reproductive number. This is all very abstract. But all you have to do is listen to different people sneeze to know that this approach makes sense.
Dr. Chun, the aforementioned South Korean doctor who studied super-spreading in the context of MERS (another coronavirus that was much more deadly, but also much harder to catch) specifically concluded that the 2015 outbreak in his country showed up the “inadequacies in the traditional [R0-based] approach,” demonstrated that SSEs played “a major role in spreading infections like SARS and MERS,” and that “the prevention and control measures for SSE should be central in controlling such outbreaks. One missed super-spreader could cause a new outbreak…By taking advantage of heterogeneity, control measures could be directed towards the smaller group of highly infectious cases or the high-risk groups.”
Of course, the law of large numbers applies to all systems. And if we were resigned to a mass spread of COVID-19 throughout our societies, it probably would be fine to fall back on traditional model, since we’d be talking about daily infection rates on the scale of many tens or hundreds of thousands, or even millions, and so individual variations would be less meaningful. But as my Quillette boss Claire Lehmann has vigorously asserted, we are very much not resigned to that; and so instead find ourselves with many countries battling to keep their symptomatic case loads in three or four figures. This is on a scale that permits SSEs to assume a large—and perhaps even dominant—role in transmission mechanics.
When COVID-19 was first declared a pandemic 16 days ago, the traditional models were useful in warning us what would happen if (literally) nothing were done to stop it. But scarcely two weeks later, we are (thankfully) a long way from nothing. Let’s go after this disease in the way that does the most good, and stop policing the paths to Pen-y-Fan.