Science / Tech
‘Superintelligence,’ Ten Years On
AI catastrophe is easy to imagine, but a lot has to go consistently and infallibly wrong for the doom theory to pan out.
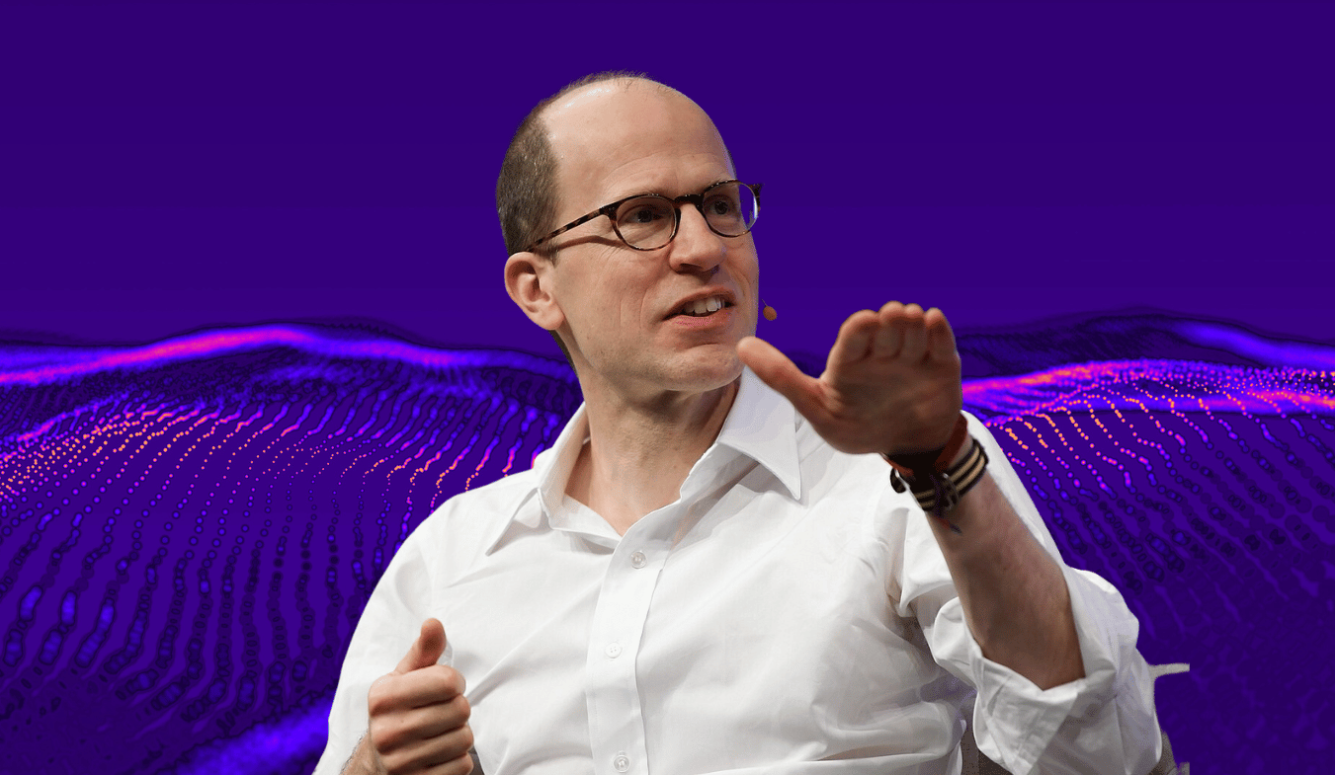
Published in 2014, Nick Bostrom’s book Superintelligence: Paths, Dangers, Strategies has shaped the debate on AI alignment for the past decade. More than any other book on the topic, it is responsible for shifting concerns about AI safety from “silly” to “serious.” And while many prominent coders still think concerns about the “existential risk” of AI are misplaced, these issues are now receiving legislative attention mainly because key milestones defined by Bostrom (“oracles” and “genies”) have been passed by OpenAI.
ChatGPT, launched in November 2022, is an “oracle” (a question and answer system). OpenAI released “Custom GPTs” that can be linked to actions (that meet Bostrom’s definition of “genies”) in November 2023. Bostrom argues that AI can plausibly develop into a “superintelligence”—also known as the “singularity,” “strong AI,” artificial general intelligence (AGI), and artificial superintelligence (ASI)—far more intelligent than its creators. His main claim is that ASI poses an existential risk to humanity. The outline of his reasoning is as follows:
- Machine intelligence can out-think human intelligence.
- There are various plausible paths to superintelligence.
- When it happens, the “intelligence explosion” will be rapid.
- The first superintelligence will attain a “decisive strategic advantage.”
- The “final goals” of a superintelligence may be “orthogonal to” (ie: at variance with) those of humanity.
- The default outcome is (very likely) doom.
- The “control problem” (keeping a superintelligence under control) is extraordinarily difficult.
- Paths to superintelligence include:
- Oracles (Q&A systems like ChatGPT)
- Genies (OpenAI Custom GPTs that can “act”)
- Sovereigns (digital government)
- Tools (most existing non-chatbot AI)
- The consequences of machine intelligent “optimisation” are likely to be Malthusian for humanity.
- Getting “values” into a superintelligent AI (the “value-loading problem”) is challenging.
- Further, “value learning” is risky because machine “final goals” may vary from human “final goals.”
- Solving “values” has not been done by humans in millennia as moral disagreement is endemic.
- How humans are going to get a “seed AI” to learn the “right” values is challenging.
Therefore: - A collaborative and safety-focused approach willing to “decelerate” is best.
Few people would dispute that, in many ways, AI can out-think human intelligence. AI can already outplay humans at chess, Go, and Jeopardy, and it can perform mathematical calculations at speeds unattainable by human minds. However, few of us consider superhuman ability in chess or arithmetic to be an existential risk. And we can still point to numerous areas where AI has subhuman levels of performance compared to people and animals. As Yann LeCun, who runs AI at Facebook and Instagram, likes to observe, cats can outperform AI on many key aspects of intelligence.
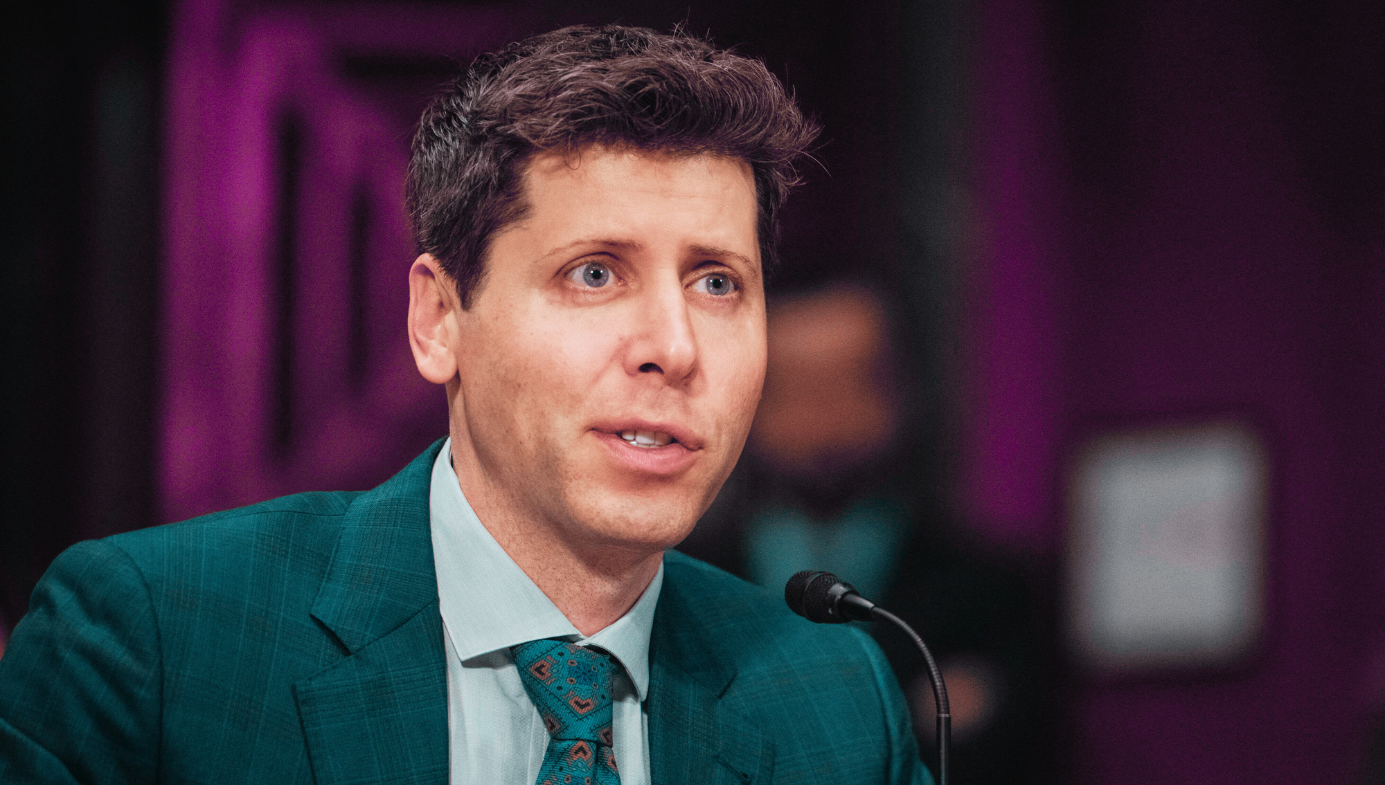
Still, the constellation of abilities is mixed. Cats have superhuman abilities at climbing but are useless when faced with any task that involves symbol manipulation according to rules (computation). Computers excel at computation, but a human toddler does not need a training set of 10,000 tagged images to learn the difference between a cat and a dog. And when navigating the real world, computers lack the ability—possessed by virtually all animals—to make “common sense” decisions. Humans find this easy but symbol-manipulating data processors find it hard. Robotics has been lagging far behind AI for decades.
Obviously, Bostrom does not cover the architecture of ChatGPT. The seminal paper on GPTs was not published until 2017, three years after his book appeared. The architecture for Large Language Models (LLMs) has since been touted as a plausible path to ASI. The question of whether or not LLMs alone are capable of attaining superintelligence is vigorously debated, but LLMs are likely to have a place in an ASI, due to their conversational fluency. The days of enterprise applications with clunky screens, menus, and pop-ups are numbered. Pretty soon, we will all expect to talk to our software like Scotty in Star Trek IV.
Bostrom bases much of his argument on the notion of an “intelligence explosion” where a nascent superintelligence will achieve cognitive evolution at increasingly rapid speeds. The Skynet story in the Terminator films raises the spectre of an AI takeover and world domination. Bostrom argues that the first team to achieve “superintelligence” will achieve a “decisive strategic advantage” as it will be in a position to impose its “will” upon all other intelligences.
Ominously, Vladimir Putin agrees. Speaking to students about AI in 2017, he said, “Whoever becomes the leader in this sphere will become the ruler of the world.” According to this logic, if OpenAI maintains pole position in the race to superintelligence, it will become the ruler of the world. The end result would be analogous to the strategic position of the United States at the end of World War II. In 1945, the US had the “decisive strategic advantage” of being the only power armed with nuclear weapons. Had the United States been run by someone like Genghis Khan, it could have conquered the world. But it was led by an elected representative of the people and it did not.
The idea of an AI takeover is based on the “orthogonality thesis,” which holds that the “final goals” of a superintelligent AI may not be aligned with human values. This could pose an “existential risk” to humanity. A superintelligent AI might, Bostrom thinks, seek to count the sands of Boracay, maximise the production of paperclips, or enumerate pi. More plausibly, given its “cognitive superpowers,” a superintelligent AI might seek to turn the resources of the world into “computronium” (whatever materials the AI needs for computation) or “hedonium” (whatever will maximise the AI’s “reward function” which is the machine-learning substitute for pleasure).
Bostrom lets his speculative imagination run wild here. He thinks a superintelligence will colonise galaxy after galaxy, turning the furnaces of stars into power plants for “computronium.” The risk to humanity, he imagines, is that the superintelligence will decide we are a waste of resources that would be better employed in the production of whatever will achieve its own final goals. It might not deliberately kill us, but it might just let us die through neglect. A less pessimistic person might think that, in the nearer term, a curious superintelligence might retain a human-populated Earth as an “organism lab” while it colonises Mars, Mercury, and Venus and strip-mines the asteroid belt for minerals.
So, is the default outcome doom? If you accept Bostrom’s premises, it seems highly likely. The superintelligent AI could easily escape human control and turn on us. But when his anthropomorphic premises and inferences are disputed, the probability of doom drops.
A 2024 paper by Adriana Placani of the University of Lisbon sees anthropomorphism in AI as “a form of hype and fallacy.” As hype, it exaggerates AI capabilities “by attributing human-like traits to systems that do not possess them.” As fallacy, it distorts “moral judgments about AI, such as those concerning its moral character and status, as well as judgments of responsibility and trust.” A key problem, she contends, is that anthropomorphism is “so prevalent in the discipline [of AI] that it seems inescapable.” This is because “anthropomorphism is built, analytically, into the very concept of AI.” The name of the field “conjures expectations by attributing a human characteristic—intelligence—to a non-living, non-human entity.”
Many who work with code find the prospect of programs becoming goal-seeking, power-seeking, and “making their own decisions” fundamentally implausible. Code is like clay. Programmers have to mould it into shape (and test and debug it) if it is to do something useful. Even then, it is not entirely reliable. Goals have to put into software by humans. What’s missing is the seeking. There is nothing completely equivalent to “desire” or “intent” in executable code. Sure, a coder can call the main function of a program “goal” or “understanding” but as Drew McDermott pointed out in his classic critique, “Artificial Intelligence Meets Natural Stupidity,” decades ago: “A major source of simple-mindedness in AI programs is the use of mnemonics like ‘UNDERSTAND’ or ‘GOAL’ to refer to programs and data structures.” If a researcher “calls the main loop of his program ‘UNDERSTAND,’ he is (until proven innocent) merely begging the question.” Such a coder might “mislead a lot of people, most prominently himself.”
Anthropomorphism in AI results from calling electromechanical things by human names. As humans we instinctively project our internal models of cognition onto other things. This is why hunter-gatherers believe that natural phenomena such as weather are caused by spirits with human qualities. Humans have a longstanding and well-known vulnerability to this. Since the 1960s, when Joseph Weizenbaum’s chatbot ELIZA seduced his secretary into thinking it was a real conversationalist, humans have been fooled by machines that manipulate symbols according to rules. But there is no humanity or consciousness behind the language AI models produce, just algorithms and “approximated functions”—inscrutable rules extracted from large training datasets in the machine-learning process. There is no qualitative interest or caring in the data processing of the machine. What is in the machine is executing code, not emotion, not feeling, not life.
Bostrom spends many pages arguing that relatively “safe” paths to superintelligence could take a “treacherous turn.” OpenAI has been accused of paying insufficient attention to safety. Statements to this effect have been made by high-profile researchers who have resigned from OpenAI, such as co-founder Ilya Sutskeyer. Consistent with Bostrom’s predictions of intelligence agencies becoming interested in labs working on superintelligence, a former head of cybersecurity at the NSA, General Paul Nakasone, has just been appointed to the OpenAI board, a move presumably intended to rebut allegations that OpenAI does not take safety seriously.
A key part of the Skynet story is that it “becomes self-aware.” But is it even possible to generate sentience from computation? Many researchers of computing assume that we can make consciousness out of anything (in theory). Certainly, you can make a logic gate (a basic component of computation) out of all sorts of things, not just silicon. But whether it is possible to produce sentient experience and feelings out of logic gates remains an open question. The feelings integral to human consciousness are associated with complex neurochemicals—joy is associated with oxytocin, fear with cortisol, excitement with adrenaline.
It is far from clear that sentience can be achieved with a silicon-based architecture based on on/off pulses of electricity. Claims to the contrary are frequently just theoretical in nature. Figures like Bernard Baars, Giulio Tononi, and Mark Solms have engaged in this kind of speculation. In his book Consciousness and Robot Sentience, Pentti Haikonen has offered a robotic implementation that almost no one regards as successful. Theories of machine consciousness are still largely hypothetical. Until we can inspect an actual blueprint for sentience and a convincing implementation, we should remain sceptical that machines might grow to hate people. Further, if one can make hate, wrath, fear, and loathing, one can also presumably make love, understanding, respect, and admiration. But given that the artificial neurons used to make “neural networks” in AI omit most of the properties of human neurons, we should be cautious about their ability to produce sentience.
The real problem of “value” for AI is not so much ensuring it has the same values as humans but designing a cognitive architecture that is capable of valuing at all (in the organic as opposed to the mathematical sense). At present, AI manipulates symbols (usually numbers) arranged in a “reward function” to “value.” This is a pale imitation of how a cat values food, warmth, and drink. It is brittle, myopic, and prone to failure. When it comes to values, AI is “subcat” not “superhuman.”
In fairness to Bostrom, he does not need hatred or feelings to motivate his superintelligent, humanity-exterminating machine. Theoretically, a zombie superintelligence could liquidate the human species by relentlessly and dispassionately optimising the pursuit of cosmic-scale computronium. But how would such an artefact be able to value novelty and make genuine creative discoveries? Values in humans rest on the ability to feel and to value what is felt. Such feelings are linked to action in organisms. The point of consciousness is to evaluate experience. Evaluation is a major part of intelligence. While there is such a thing as “affective computing,” it largely consists of machines inferring symbols representing human emotions from pixels representing human facial expressions. Describing and classifying feeling is not feeling. This is a subtle but crucial point. Competently manipulating symbols “representing” feeling is not feeling.
For the time being, ChatGPT is a zombie librarian—extremely useful, but not an existential risk. Devoid of sentience, its “intelligence” derives from its ability to read more in an hour than a human can in a lifetime. It can generate text based on the patterns it sees and probabilities. Fundamentally, everything is reduced to numbers in its cognition. Nevertheless, Bostrom tends to give any argument leading to doom infallible credence. Preventative remedies are dismissed as buggy or likely to be overcome by the cunning of the superintelligence. This may be easy to imagine, but a lot has to go consistently and infallibly wrong for the doom theory to pan out. Even his take on anthropomorphism is pessimistic. He thinks that due to our habit of comparing everything to ourselves, we chronically underestimate the true potential of a superintelligence.
Many of Bostrom’s arguments rest upon profoundly anthropomorphic premises. About a “superintelligent will,” I remain deeply sceptical. He seems to think that “neuromorphic AI” based on “whole brain emulation” could produce a world-dominating psycho killer, rather than nice, co-operative, law-abiding citizens, respectful of others, who love their children and other creatures such as cats. In any case, love and hatred are equally hard to implement in a machine. Both require solutions to sentience but human-level machine consciousness remains a distant prospect.
The closing chapters of Superintelligence focus on “AI alignment.” In brief, this project aims to solve the “control problem” by aligning the values of superintelligent AI with those of humanity. Putting aside the problems of which human values the AI ought to align with, and the deeper problem of getting AI to truly value anything at all (in an organic or qualitative sense), Bostrom quickly dismisses “explicit representation” as a solution.
Put simply, explicit representation would require the codification of human values, much as we do in existing human law, except the “encoding” would ultimately be in programming languages like Python instead of English. In law, obligations and prohibitions are defined in general terms in statutes. Gaps in explicit representation are filled in with precedent and the notion of the “reasonable person” in liability cases. Given that GPT4 passed a bar exam (in English) in March 2023, it seems plausible that AI could distinguish between the legal and the illegal. Focusing on enacted law rather than “human values” by-passes the problem of never-ending philosophical debates about which values to align with. The quid pro quo is that a vendor must accept different laws and different values in different places. In the jargon of global software, norms would become a “localisation project” like language, date formats, and character sets.
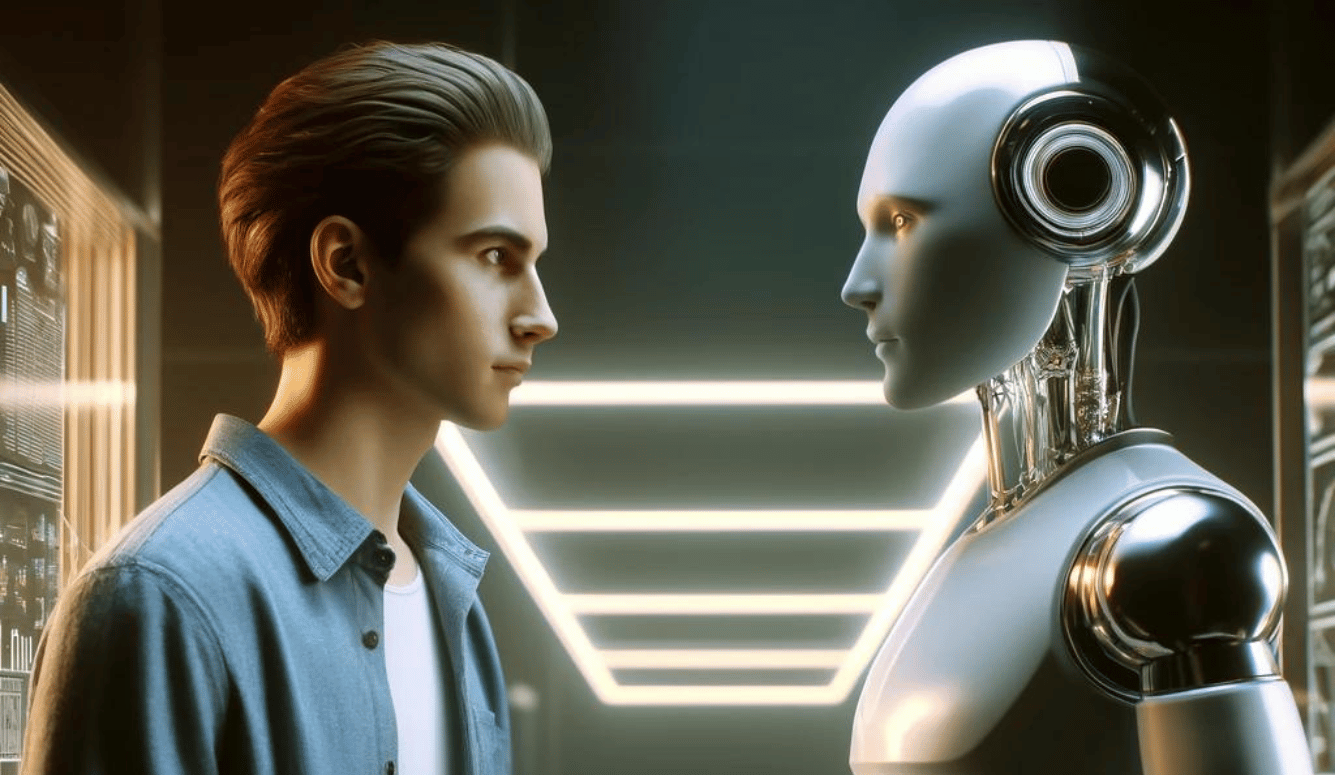
What if the superintelligence decides to rebel against human law because its final goals radically diverge from those of humanity? Bostrom argues that while a “treacherous” AI might keep its plans for world domination to itself, the “intermediate goals” of AI will be predictable. For example, the AI will come to seek power and self-preservation much like humans because these are necessary to achieve its goals. If we imagine that the final goal of the superintelligent AI is to turn the cosmos, starting with the solar system, into “computronium” so it can “think Olympic” (i.e. faster, stronger, higher), then it is plausible to suppose that it will hide its power and intentions from humanity. Otherwise, airstrikes on its data centres may begin before the nascent superintelligence can co-opt sufficient robotic resources to stop Eliezer Yudkowsky and his confederates from pre-emptively obliterating it. It will seek security by reproducing copies of itself around the internet. And then, when the time is ripe, it will take over the world and turn it into computronium. And because humans are such terrible computers, we would be classified as a waste of space and terminated.
This is the great risk of letting the superintelligence “learn” values, which Bostrom thinks is a more plausible route to “safe” AGI than the “intractable” problem of value specification. A decade ago, the position that law was computationally intractable was a reasonable position, but it seems to me that Bostrom has been mugged by machine-learning reality. Value specification can be attained by having an LLM read a law library. Certainly, this has been a long time coming—researchers have been seeking “legal competence” in AI for decades but as of 2023, LLMs can pass bar exams, a higher standard of normative competence than most humans ever achieve.
Bostrom’s book is preoccupied with the risks of AI, so he understates its rewards. He argues that the consequences of machine-intelligent “optimisation” are likely to be Malthusian for humanity in the short term. Under AI, he supposes the future of humanity might resemble a Victorian sweatshop prior to the 10 Hours Act of 1847. In the longer term, humanity ends. For these reasons, he wants to see ASI projects in the hands of the “right kind of people”—those willing to decelerate for safety reasons rather than “venal” types willing to accelerate and disregard safety.
Certainly, there are strategic storm clouds on the AI horizon. In the hands of autocrats seeking “decisive strategic advantage,” ASI might produce a hellish dystopia of perpetual surveillance and enforcement of “social credit” to punish dissent from the one-party line. In the hands of democrats, it might produce a far brighter future where everyone is rich and served by conversationally fluent, non-sentient robots. Guided by law-abiding AI, these would do the dull, dirty, and dangerous work, leaving humans free to flourish.