Science / Tech
Autonomous Vehicles: Hype or Reality?
AVs are essentially robots with the same requirements as human-driven vehicles—driving and parking skills, the ability to communicate with other cars and the infrastructure, navigation skills, and access to a source of energy.
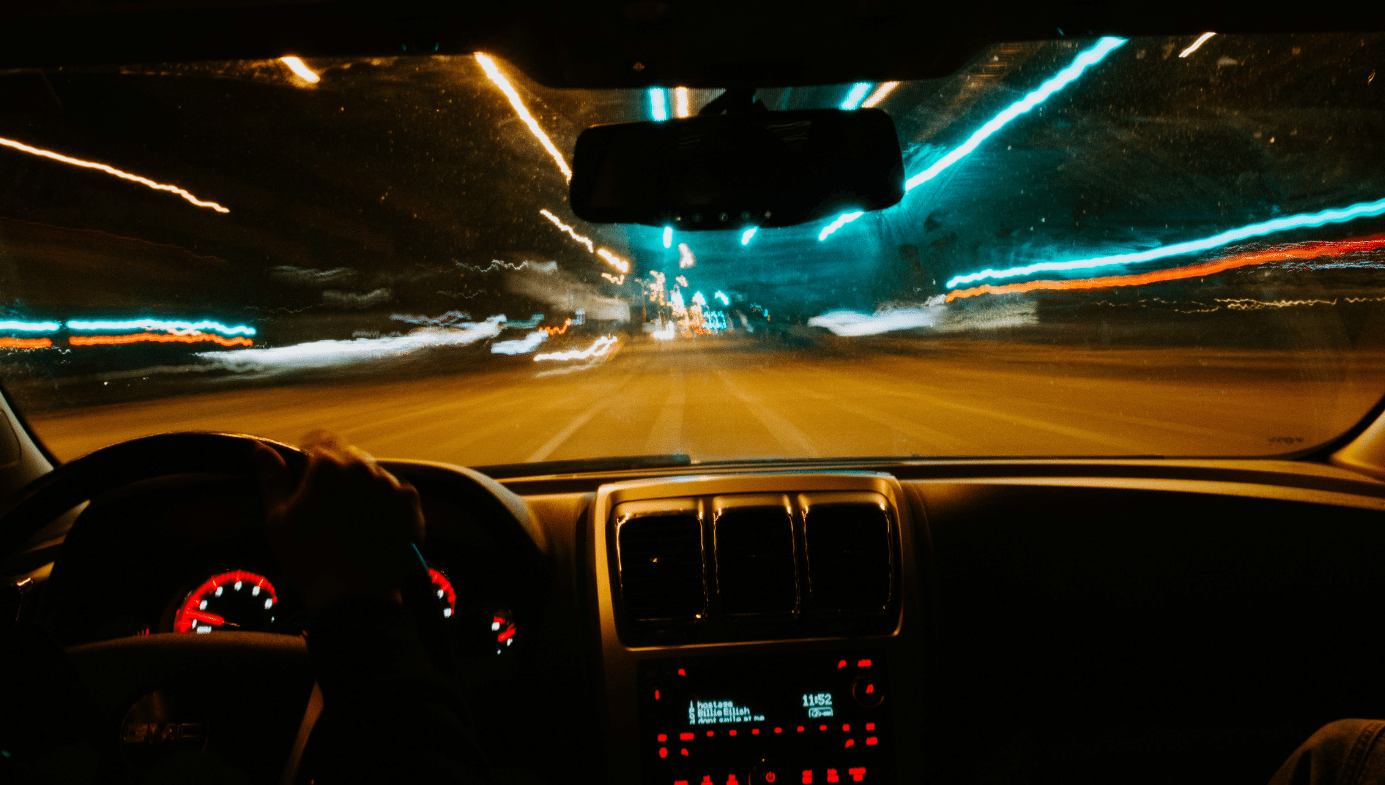
Automobile accidents are the second leading cause of death worldwide, with roughly 1.3 million fatalities annually according to the World Health Organization. China accounts for roughly 260,000 of those and the United States roughly 40,000. Car ownership is growing in developing countries, which will increase the number of fatalities globally. The National Highway and Transportation Safety Agency found that human error was the probable cause in over 92 percent of the cases in the US. Some estimates suggest that replacing human drivers with autonomous vehicles (AVs) could eliminate as many as a million global fatalities annually.
People have become dependent on truck drivers to deliver the goods we use on a daily basis. There is currently a shortage of truck drivers and many are nearing retirement. So, we can expect to see alternative modes of transportation in the commercial sector as well. Deliveries are a challenge in cities like Amsterdam where water covers over 20 percent of its surface area—its waterway system of over 60 miles of canals is a main source for transportation. Amsterdam is also heavily congested with 2.5 million people and over 3.5 million visitors annually. The city has teamed up with researchers from MIT to test autonomous boats to address its geographical and density issues. Amsterdam could potentially use these prototypes as a delivery service as well as for waste collection and water taxis to reduce traffic congestion.
Finally, internal combustion engines are a major source of greenhouse gases. For decades, climate change and urban living conditions have led to discussions of phasing them out and replacing them with shared AVs. This would help to reduce traffic congestion that wastes time and fuel. The Council of Economic Advisers estimates that congestion costs the US economy more than $120 billion annually.
AVs are essentially robots with the same requirements as human-driven vehicles—driving and parking skills, the ability to communicate with other cars and the infrastructure, navigation skills, and access to a source of energy. AI is involved in training vehicles to drive and park, reading road signs, and detecting objects on the highways and then processing them to make decisions. Will society adopt electric AVs on the land, sea, and in the air to remedy a myriad of social problems?
Object detection
Utilizing natural language-processing, AVs are able to read road signs, but they must then make decisions about how to proceed. If the message is not clear the second step can create problems because deep learning is still in the early stages of acquiring reasoning and common sense.
Detecting objects—lanes, pedestrians, bicycles, animals, debris, other vehicles, and so on—is more complicated. Training AI to detect an object from one angle is costly and requires massive amounts of data. The actual data is the edge of an object, and the AI is trained to detect patterns until a desired accuracy rate is reached. For AI systems, it is impractical to even attempt developing software for detecting every object from every possible angle. The human brain, on the other hand, has evolved to recognize objects whether they are large or small, made of different materials, or seen from a front, back, or side view.
Computer vision utilizes ultrasonic sensors and cameras, which have limitations of depth perception, and in some weather and light conditions. So, they are complemented by more expensive technologies including radar, which is reliable in a wide range of weather conditions, and lidar, which is good at recognizing small objects like rocks and cyclists, and mapping 3D objects.
Decision-making
According to Polanyi’s paradox, certain skills—such as riding bicycles, playing chess and Go, and driving cars—aren’t suited to learning by instructions. Once an AV detects an object, programming rules for responding to it will only work if you can anticipate all possible scenarios. However, it is too complex to write a rule for every possible situation. Instead, AVs learn from experience to figure out how to make decisions and navigate on their own. For example, the software for AVs merging on highways and at intersections isn’t programmed. Using reinforcement learning, the cars learn which merging techniques were unsuccessful and which ones went smoothly and they update instructions with each experience.
One option is for AVs to learn how to make decisions through simulations. As of 2020, Waymo (formerly the Google self-driving car project and a subsidiary of the parent company Alphabet) has simulated 15 billion miles of driving compared to just 20 million miles of actual driving. Through simulations, self-driving cars are gaining experience and learning more about possible scenarios they might encounter. Unfortunately, the process of machine learning cannot tell which models in the simulation will work because of the differences between the data on which the AI was trained and that in the real world.
Another option is to test cars on Autonomous Vehicle Proving Grounds designated by the federal government. These are custom-built with highways, parking lots, intersections, and communication networks where vehicles “talk” to each other and to the infrastructure. AI software, underlying processing hardware, and sensors are responsible for decision-making in AVs. AI software is continually undergoing development, and each updated version needs testing.
Since the current Level 2 and 3 AVs require human driver supervision on highways, in many cases they are not allowed to make autonomous decisions there. These test tracks are designed to expose AVs to as many commonly encountered and edge cases, issues that occur in extreme conditions, and driving situations as possible. AV proving grounds provide real world conditions, but are much more expensive than simulations.
AVs obtain a learner’s permit
The extent to which AI is allowed to make autonomous decisions on the highways is a work in progress. SAE International has designed a system for classifying levels of driving automation from 0 to 5. Currently, AVs essentially have a learner’s permit, but before AVs are allowed drive on the roads unassisted, they will have to gain public acceptance.
Levels of Automation
Level 0: No automation |
Features that may momentarily take over control of the vehicle—antilock brakes and electronic stability control—but do not have sustained control of the car. |
Level 1: Driver assistance |
A few functions such as automated cruise control and park assist. |
Level 2: Partial automation |
The driver, while always monitoring the car, does not have to steer or brake or accelerate under some driving conditions. |
Level 3: Conditional automation |
The vehicle can drive mostly autonomously, but the driver must always be alert to take over as needed. |
Level 4: High automation |
The vehicle can operate fully autonomously but is restricted to specific roads or conditions. |
Level 5: Full automation |
The vehicle can operate fully autonomously without any human driver interaction. |
Most new cars sold today are Level 1 with features such as automated cruise control and park assist. A number of companies including Tesla, Uber, Waymo, Audi, Volvo, Mercedes-Benz, and Cadillac have introduced Level 2 vehicles with automated acceleration and braking and are required to have a safety driver in the front seat available to take over if something goes wrong.
As Level 2 AVs gain experience on the highways with human drivers, they occasionally have crashes. The National Transportation Safety Board (NTSB) released its final reports on two fatal crashes involving Tesla vehicles using Autopilot. The agency determined that the main causes were unrelated to Tesla, but that Tesla’s Autopilot was a contributing factor—the driver’s inattention due to over-reliance on automation resulted in a failure to react.
Levels 3 and 4 can drive mostly autonomously, but with restrictions. Waymo has a fleet of hybrid cars in Phoenix, Arizona, that it is using to test and develop Level 5 technology specifically to pick up and drop off passengers.
Data processing and communications
Most data processing today is performed using von Neumann architecture, where the data memory and the processor are in two different places. Today, that typically means cloud computing. When cameras and sensors generate data to detect objects on the roads, processors will need to rapidly analyze the data and make real-time decisions regarding acceleration, braking, and steering. But this ability is compromised by latency issues.
One solution to latency is moving processing and data storage closer to where it is needed to improve response times. Edge computing, for example, places the processor at the site where the data is generated. Most new human-driven vehicles contain anywhere from 30 to 100 electronic control units (ECUs) that process data and control electrical systems in vehicles. These embedded systems, typically in the dashboard, control different applications such as airbags, steering, brakes, etc. ECUs process data generated by cameras and sensors in AVs and make crucial decisions on how they operate.
Since communications are sometimes unreliable, AVs are also utilizing sensor fusion or redundant systems in the event that one system malfunctions. For example, if a car’s sensors malfunctioned, it could rely on GPS technology which uses satellites to navigate safely.
Similar to bacteria, fish, and birds using swarm intelligence, self-driving cars can also benefit by communicating with each other for navigation. Researchers are currently investigating fog computing which places servers along highways for faster and more reliable navigation and for communicating data analytics. Similar to cloud, fog is a meteorological metaphor derived from a cloud close to the ground.
The US government is dedicating bandwidth for vehicle-to-vehicle and vehicle-to-infrastructure communications. Dedicated short-range communications (DSRC) have bandwidths specifically dedicated to two-way communications for critical safety applications. 5G cellular networks are another solution to latency and have broader capabilities. AVs can send data about weather conditions and traffic jams to a server, and AVs can adapt their routes accordingly. Based on these capabilities, 5G could serve as the main service while DSRC could act as a back-up service.
Batteries and charging systems
For the adoption of electric vehicles (EVs), researchers will have to find ways to make batteries cheaper and lighter, and increase their range. While most electric batteries have a range of 200–260 miles, the Tesla S achieves 370 miles, but the weight of the lithium-ion battery pack is 1,200 pounds. So, there is a trade-off between price and weight, on one hand, and more frequent charging, on the other.
Researchers are investigating several alternatives. Battery swapping is too expensive and impractical. Charging EVs requires cables, and for AVs it requires a robot or person to plug them into the car and the charging station. Solid state batteries, which have solid components, can store more energy and charge more quickly, but they are still in development.
Researchers are also investigating more efficient ways of charging batteries. Wireless charging systems provide several advantages. They would reduce the need for charging stations and the size of the battery pack, which also reduces the cost and weight of the vehicle. Wireless transfer of electrical energy requires two magnetic coils. One of the coils is located in the ground and is connected to a power source. The other coil is installed in the vehicle and is connected to the charging system and the battery of the vehicle. Energy is transferred through a magnetic field between the two coils. The technology is still in development because the energy transfer between magnetic coils needs a higher efficiency rate.
Google and Qualcomm are developing a system with which cars can recharge while driving by embedding strips of charging coils in the highway. Qualcomm has already proved that charging while driving is possible, even while the vehicle is traveling at up to 70 mph and when the surface is covered by water. Using this method, the batteries don’t refill, they just don’t lose any power while charging on the road. This would eliminate concerns about range and possibly make electricity the standard fuel for vehicles. However, the health effects of long-term exposure to weak magnetic fields are presently unclear. So, more research in this area is required to ensure safety.
A new business model for AVs
EVs are adopted in areas where drivers are willing to purchase them and regions are willing and able to support them. Norway, China, and California have developed successful models for supporting EV manufacturers and consumers. China, Europe, and the United States each have over a million electric and plug-in hybrid vehicles, and China, which has the largest car market, reached the milestone first. Norway leads the European countries and has the highest market penetration per capita in the world. The Norwegian government’s incentives include waiving taxes, public parking fees, and tolls. Consumers in California, the largest market in the United States, have benefited from state and federal tax credits.
In the United States, the standard business model for selling cars dates back to the 1930s. State laws have prohibited car manufacturers from selling their products directly to consumers or providing after-market service. Instead, state governments have mandated that customers work with a middleman for sales and service. This has prevented the Big Three automakers from having too much power over small dealerships or competing with them. However, several trends indicate that the time has arrived for developing a new business model for electric AVs. Ideally, a new business model will evolve according to what is in the best interest for all the parties involved—the states, manufacturers, dealerships, and customers.
Elon Musk determined that the independent dealership system was not compatible with the customer education and sales experience that Tesla had in mind. Musk argues that its products are different, and only Tesla can adequately support and service them. Rather than having a network of independent franchised dealerships, Tesla adopted a direct-to-consumer sales and service model. While some states are beginning to allow direct-to-consumer sales on a limited basis, many still have restrictions. So, Musk is lobbying state by state and is using loopholes such as placing a support center on an Indian reservation in New Mexico.
EVs cost roughly $12,000 more to build than comparable gasoline-powered models, and currently batteries can account for one third of the price, according to McKinsey & Company. Combined with the added expense of automation, some Tesla models are still too expensive for many consumers, even with government subsidies for EVs. Analysts estimate that bypassing the middleman could cut a vehicle’s cost to customers by roughly eight percent. That’s $800 savings for each $10,000 spent.
With the Tesla business model for EVs and electric AVs, states and dealerships will receive less revenue. With independent dealers, states typically receive a six percent sales tax on each vehicle sold. EVs reduce dealership profits and incentives to sell them because they require less service than gasoline models. However, Musk says he is willing to shift to a hybrid business model once the electric AV market is more established.
Some consumers are concerned that the direct-to-consumer business model will hand Tesla a monopoly, but Tesla has competition from other electric car manufacturers. To become more competitive, Musk has said he intends to manufacture more cars in the $25,000 range.
Tesla showrooms are not allowed to give pricing information. Rather, they direct customers to their website and business is transacted via the Internet. In 2020, millennials topped baby boomers for the first time as new car buyers, and these younger, Internet-savvy consumers generally dislike car dealerships. Tesla’s business model will certainly provide customers with a different car-buying experience—test drives may become a thing of the past, and it is hard to put a price on not having to visit car dealerships and haggle.
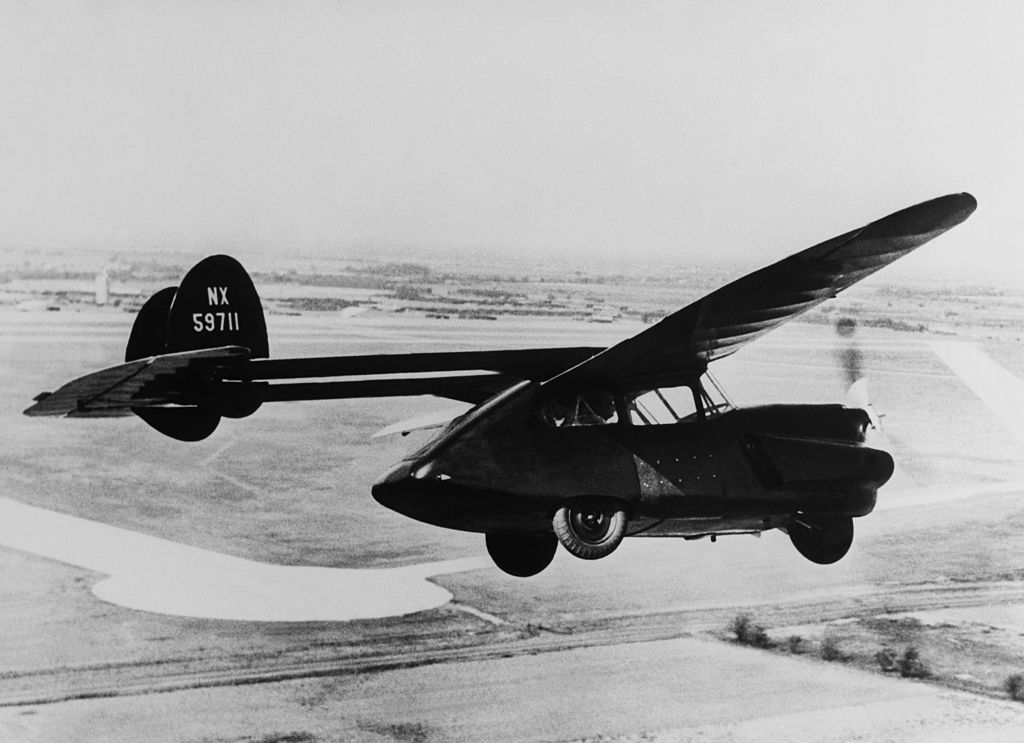
Public acceptance
For both human drivers and self-driving cars, situations arise where it is necessary to make life-and-death decisions. For example, should an AV swerve to strike and kill pedestrians, or do nothing resulting in the deaths of a vehicle’s passengers instead?
Through an interactive website called Moral Machine, MIT computer scientist Iyad Rahwan surveyed participants about moral dilemmas in different scenarios. The respondents in the survey included over two million participants from 233 countries and territories which represented various religions, countries, and cultures. The study found that the rules for morality are not universal, and not everyone values human lives or the status of children and the elderly equally.
Despite the fact that many researchers believe self-driving cars will ultimately become safer in the long-term due to a reduced number of human errors, a 2018 Reuters/Ipsos poll suggests that society is not ready to adopt AVs. Without experience or much knowledge about AVs, only 27 percent of respondents—38 percent of men and 16 percent of women—said they would feel comfortable riding in a driverless car. While younger respondents are generally more enthusiastic about AVs, 54 percent of drivers would feel less safe while sharing the road with them.
Furthermore, self-driving cars are essentially Internet-of-Things devices that utilize computers and sensors which make them vulnerable to hacking. Through the Internet or vehicle-to-vehicle communications, hackers can provide inaccurate information and confuse a car or even take over its controls. For example, hackers used “Toyota’s collision avoidance system to apply brakes on a Prius, Jeep Cherokee’s cruise control to accelerate, and the Jeep’s automated parking system to turn the steering wheel by tricking the car into thinking that it was parking itself, even though it was travelling at 80 miles per hour.” To avoid issues of this kind, machine learning experts are developing algorithms to detect unusual commands and malware activities to alert drivers.
As for environmental benefits, while AVs may have zero carbon emissions, if the charging stations powering these cars are powered by fossil fuels, the benefits are not as attractive. Also, obtaining many of the metals, including lithium, needed to build electric car batteries requires vacuuming patches of the deep ocean floor or mining on land, and environmentalists are concerned that large-scale mining could destroy entire ecosystems. In addition, a study by Boston Consulting Group found that AVs could actually increase congestion in some cities in the longer term.
Adoption or a third AI Winter?
The development of emerging technologies does not follow a linear path. Instead, they follow an S-curve of hype and failure. The enabling technologies and supporting infrastructure for AVs have multiple S-curves. AI has experienced two hype cycles since the 1950s, which resulted in two AI Winters with reduced funding and public interest. Since AVs utilize multiples technologies, predicting the development of AVs is an extremely complex task. Instead, technology analysts like me forecast probable scenarios based on trends.
The worst-case scenario for AVs is that they don’t make it through the initial technological hurdle. If Musk dropped off 500,000 AVs in your hometown, everything would not run smoothly because the current transportation infrastructure is designed for human drivers, not driverless cars. If roads are not well marked, AVs will not be able to navigate lanes safely. Also, current vehicle-to-vehicle and vehicle-to-infrastructure communications, real-time data processing, and battery and charging technologies will not support a large number of AVs adequately. However, without the first step, the infrastructural and regulatory hurdles are irrelevant.
While technologies for AVs such as navigation and natural language processing are incrementally progressing towards automation, others still have a long way to go. The hardware and software for computer vision and decision-making are in the developmental stages. AI researchers including NYU’s Gary Marcus warn that if we overhype the AI necessary for AVs, we could experience a Third AI Winter.
Seven decades after the Dartmouth conference that launched AI, and two AI Winters later, we are still working through issues of narrow AI, causality, understanding neuroscience, and common sense. We are now in the Third Wave of AI which is focused on the limitations of deep learning.
Despite these technological hurdles, there is currently optimism that AI researchers will eventually resolve them. Funding by DARPA and a number of human brain initiatives specifically target the limitations of deep learning and a better understanding of human brain complexity. The automobile industry and venture capitalists—primarily in the US, China, Germany, the United Kingdom, and Israel—have invested billions of dollars in technologies related to AVs. The Chinese government has set a goal for 10 percent of vehicles reaching Level 4/5 autonomy by 2030, which could elevate rivalry with Silicon Valley and stimulate the innovation necessary for adoption. In time, the necessary breakthroughs in AI and battery technology should pave the way for infrastructural support and regulations necessary for the adoption of AVs. And when they do, public acceptance will likely follow.