AI Debate
What Computer-Generated Language Tells Us About Our Own Ideological Thinking
Thus, the ancient question of what separates humans from animals is the inverse of the more recent question of what separates humans from computers.
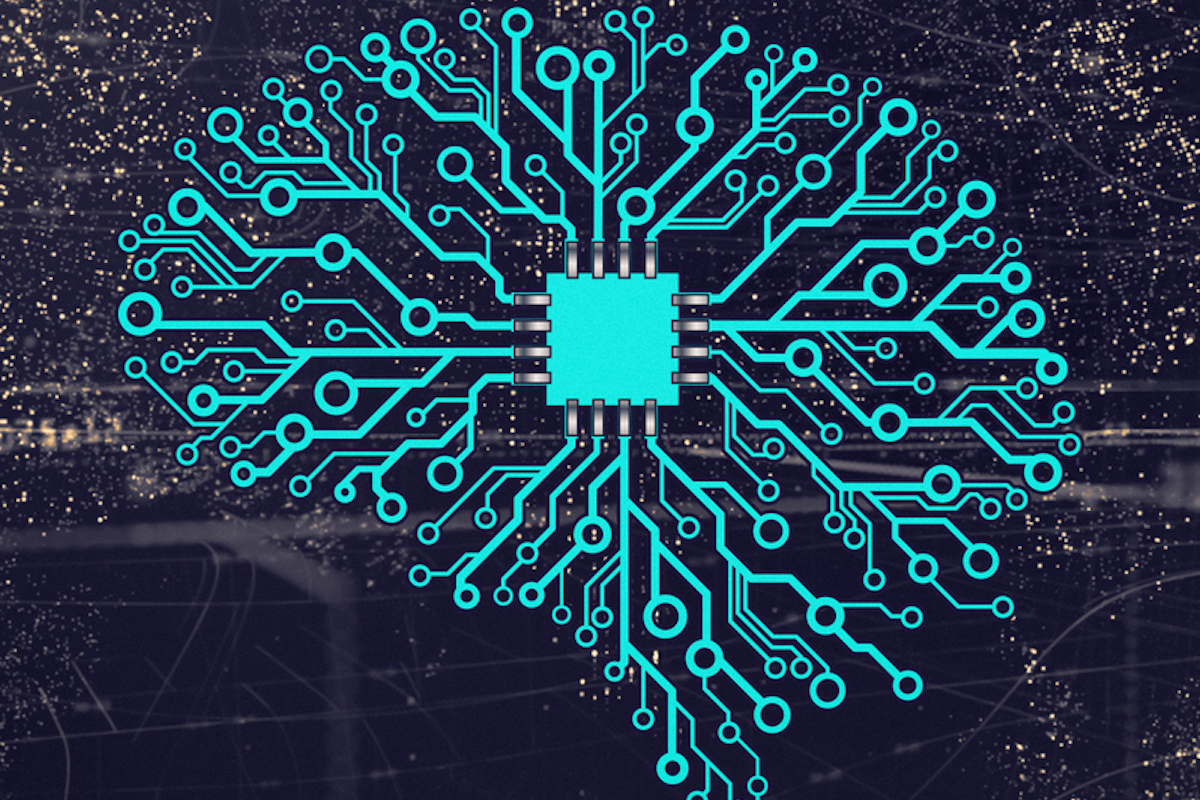
Earlier this year, the San Francisco-based artificial-intelligence research laboratory OpenAI built GPT-3, a 175-billion-parameter text generator. Compared to its predecessor—the humorously dissociative GPT-2, which had been trained on a data set less than one-hundredth as large—GPT-3 is a startlingly convincing writer. It can answer questions (mostly) accurately, produce coherent poetry, and write code based on verbal descriptions. With the right prompting, it even comes across as self-aware and insightful. For instance, here is GPT-3’s answer to a question about whether it can suffer: “I can have incorrect beliefs, and my output is only as good as the source of my input, so if someone gives me garbled text, then I will predict garbled text. The only sense in which this is suffering is if you think computational errors are somehow ‘bad.'”
Naturally, this performance improvement has triggered a great deal of introspection. Does GPT-3 understand English? Have we finally created artificial general intelligence, or is it just “glorified auto-complete”? Or, a third, more disturbing possibility: Is the human mind itself anything more than a glorified auto-complete?
The good news is that, yes, the human mind usually acts as more than just an auto-complete. But there are indeed times when our intelligence can come to closely resemble GPT, in regard to both the output and the processes used to generate it. By developing an understanding of how we lapse into that computer-like mode, we can gain surprising insights into not just the nature of our brains, but also into the strains imposed by ideological radicalism and political polarization.
* * *
One common way of dismissing GPT’s achievement is to categorize it as mere prediction, as opposed to true intelligence. The problem with this response is that all intelligence is prediction, at some level or another. To react intelligently to a situation is to form correct expectations about how it will play out, and then apply those expectations so as to further one’s goals. In order to do this, intelligent beings—both humans and GPT—form models of the world, logical connections between cause and effect: If this, then that.
You and I form models largely by trial and error, especially during childhood. You try something—ow, that hurt—and that lesson is stored away as a model to influence future, hopefully more intelligent, behavior. In other cases—hey, that worked pretty well—the mental pathways that led to an action get strengthened, ready for use in similar situations in the future.
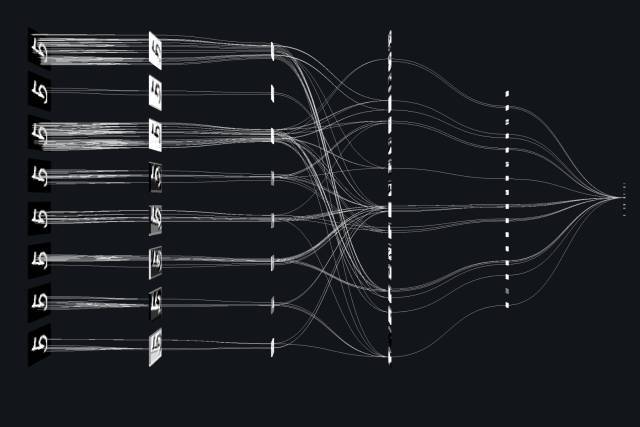
The ersatz-childhood, or training phase, of a neural network such as GPT is similar: The software is confronted with partial texts and asked to predict the missing portion. The more closely the result corresponds to the right answer, the more the algorithm strengthens the logical rules that led to that result, a process called backpropagation. After chewing on many billions of words of training text, GPT’s model of what a text ought to look like based on a new prompt is, apparently, pretty good. Fundamentally, the human mind and GPT are both prediction machines. And so, if we are to reassure ourselves that there is something essentially different about our human intelligence, the difference must lie in what is being predicted.
* * *
The philosopher John Searle once posed a thought experiment called “The Chinese Room” to illustrate his argument that human intelligence is different than AI. Imagine a man locked in a room full of boxes of paper and a slot in the wall. Into the slot, taskmasters insert sentences written in Chinese. The man inside does not read Chinese, but he does have in front of him a complete set of instructions: Whatever the message passed to him, he can retrieve an appropriate slip from the boxes surrounding him and pass it back out—a slip containing a coherent reply to the original request. Searle argues that we cannot say the man knows Chinese, even though he can respond coherently when prompted.
A common response is that the room itself—the instruction set and the boxes of replies—“knows” Chinese, even if the man alone does not. The room, of course, is the GPT in this metaphor. But what does it mean to know a language? Is it sufficient to be able to use it proficiently and coherently, or should there be some sort of deeper semantic understanding? How would we determine if the GPT “understands” the input and output text? Is the question even meaningful?
One important clue is that the history of computing, in an important sense, has moved in a direction opposite to the development of the human mind. Computers began with explicit symbolic manipulation. From the modest but still near-instantaneous arithmetic tasks performed by the first computers, to the state-of-the-art 3D video games that max out the processing power of today’s graphics units, programming is an exercise in making processes entirely explicit. As an old programming adage goes, a computer is dumb, and will do exactly what you tell it to, even if it’s not what you meant to tell it.
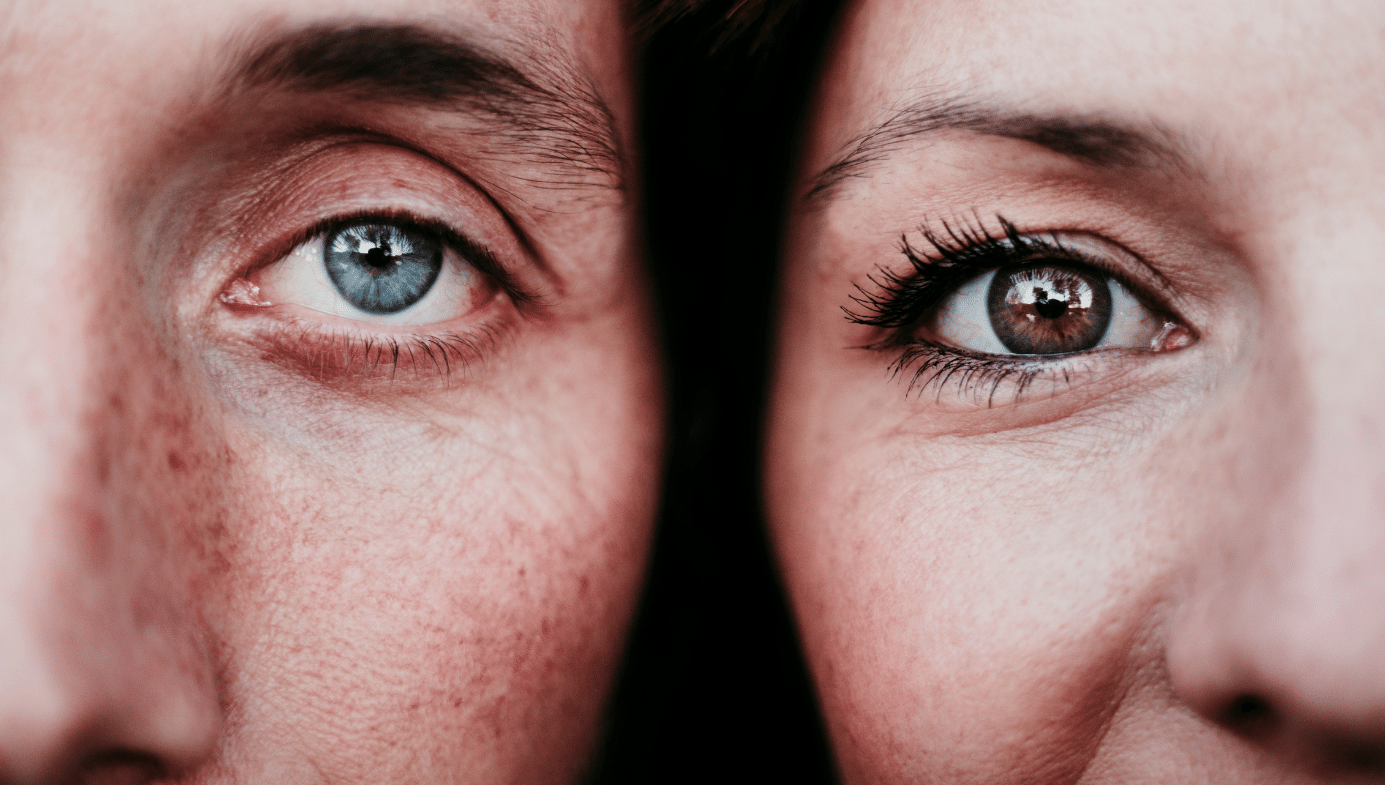
Though analytic philosophers and some early AI pioneers thought of intelligence as consisting in this kind of logical-symbol manipulation—the kind of thing computers excelled at from the beginning—humans actually develop this ability at a later stage in learning. In fact, when humans do manage to develop logical symbol manipulation, it is a skill co-opted from the language faculty; a simulation layer developed on top of the much looser sort of symbol manipulation we use to communicate with one another in words and gestures. Even after years of training, there is no savant on the planet who could outperform a basic calculator doing arithmetic on sufficiently large numbers. Rationalism is an extremely recent refinement of the language faculty, which itself is (in evolutionary terms) a relatively recent add-on to the more basic animal drives we share with our primate cousins.
Thus, the ancient question of what separates humans from animals is the inverse of the more recent question of what separates humans from computers. With GPT, computers have finally worked backward (as seen in animal terms), from explicit symbol manipulation to a practically fluent generative language faculty. The result might be thought of as a human shell, missing its animal core.
This piece, missing from GPT as well as from Searle’s Chinese Room, is the key to understanding what distinguishes human intelligence from artificial intelligence.
* * *
The guiding imperative of any living organism, no matter how intelligent, is: survive and reproduce. For those creatures with a central nervous system, these imperatives—along with subgoals such as procuring food, avoiding predators, and finding mates—are encoded as constructs we would recognize as mental models. Importantly, however, the mental models are always action-oriented. They are inextricably bound up with motivations. The imperative comes first; the representations come later, if at all, and exist only in service of the imperative.
Human language is an incredibly powerful model-making tool; significantly, one that allows us to transmit models cheaply to others. (“You’ll find berries in those trees. But if you hear anything hiss, run quickly.”) But human motivation is still driven by the proximate biological and social goals necessary to ensure survival and reproduction. Mental models don’t motivate themselves: As anyone who has fallen asleep in algebra class wondering “When will I ever use this?” knows, learning must typically be motivated by something outside the content of the knowledge system itself.
Language is not, of course, the only tool we have to build models. In the course of growing up, humans form many different kinds. Physical models let us predict how objects will behave, which is why little league outfielders can run to where a ball is hit without knowing anything about Newtonian mechanics. Proprioceptive models give us a sense of what our bodies are physically capable of doing. Social models let us predict how other people will behave. The latter, especially, is closely bound up with language. But none of these models are essentially linguistic, even if they can be retroactively examined and refined using language.
This, then, is the fundamental difference between human intelligence and GPT output: Human intelligence is a collection of models of the world, with language serving as one tool. GPT is a model of language. Humans are motivated to use language to try to predict features of the social or physical world because they live in those worlds. GPT, however, has no body, so it has no proprioceptive models. It has no social models or physical models. Its only motivation, if that word even fits, is the extrinsic reward function it was trained on, one that rewards prediction of text as text. It is in this sense alone that human intelligence is something different in kind from GPT.
* * *
Humans should not, however, be too quick to do a victory lap. We can easily fall into the trap of modeling language rather than modeling the world, and, in doing so, lapse into processes not unlike those of GPT.
Extrinsically motivated learning, for instance—the kind you do because you have to, not because you see any real need for it—can lead to GPT-like cognition in students. Economist Robin Hanson has noted that undergraduate essays frequently fail to rise above the coherence of GPT output. Students unmotivated to internalize a model of the world using the language of the class will often simply model the language of the class, as with GPT, hoping to produce verbal formulations that result in a good grade. Most of us would not regard this as successful pedagogy.
The internalization of an ideology can be thought of similarly. In this case, a person’s model of the social world—along with the fervent motivation it entails—gets unconsciously replaced, in whole or in part, by a model of language, disconnecting it from the feedback that a model of the world would provide. We stop trying to predict effects, or reactions, and instead start trying to predict text. One sees this in political speeches delivered to partisan crowds, the literature of more insular religious sects and cults, or on social media platforms dominated by dogmatists seeking to gain approval from like-minded dogmatists.
What makes the process so seductive is that it can give the same jolt of insight we experience when we truly do correctly model the real world of objects, people, and feelings. If I can generate convincing text, it must mean I really understand the system I’m trying to predict—despite the fact that the resulting mental model is largely self-referential. When a community then finds itself at a loss to defend against the weaponization of its values, it becomes embroiled in the piety-contest dynamic I’ve described in a previous Quillette article: “a community in which competing statements are judged, not on the basis of their accuracy or coherence, but by the degree to which they reflect some sacred value.”
There exists, for instance, a set of rote formulas by which nearly anything can be denounced as “problematic” vis-à-vis social-justice orthodoxy: Just invoke the idea of power structures or disparate impact. Similar formulas can be used by a religious zealot to denounce heresy, by a nationalist to denounce an opponent as treasonous, or—in principle—by someone committed to any sacred value to denounce anything. Vestiges of a mental model of the social world might allow adherents to reject certain extreme or self-defeating uses of the formula—for example, Dinesh D’Souza’s repeated and failed attempts to flip prejudice accusations—but the process has no inherent limit. As the mental model becomes more purely linguistic, self-referential, and unmoored from the social and physical referents in which it was originally rooted, the formula in itself gains more and more power as an incantation.
This kind of purely linguistic cognition can be done easily enough by a computer now. When we run all experience through a single mental model, motivated by a single sacred value, that’s when we’re most vulnerable to the piety contest dynamic. Avoiding it doesn’t require us to stamp out our sacred values, only that we acknowledge multiple sacred values that motivate multiple mental models.
Just as a mental model cannot motivate itself, it also cannot change itself, or notice when it’s degenerated into something circular. We can only check our mental models and confront them with the real world using other mental models with independent motivations. A commitment to justice, for example, must be tempered by a commitment to mercy—and vice versa. A commitment to an abstract ideal must be tempered by a commitment to empirical truth, and vice versa. Any of these, on their own and unchallenged by an opposing value, has the potential to devolve into a self-referential fundamentalism.
Thus, in our current polarized moment, in the grip of a still-intensifying piety contest, it is critical to avoid the temptation to retreat into our own communities where we can confirm our own values and drown out the feedback from others. When one sacred value dominates and subsumes all others, when all experience is filtered through one mental model—that’s when the human mind really does become a form of “glorified auto-complete,” sometimes with monstrous results.