Top Stories
Brotopia—Analysis and Review
Chang shows no awareness that gender parity is the exception not the rule in the US workplace.
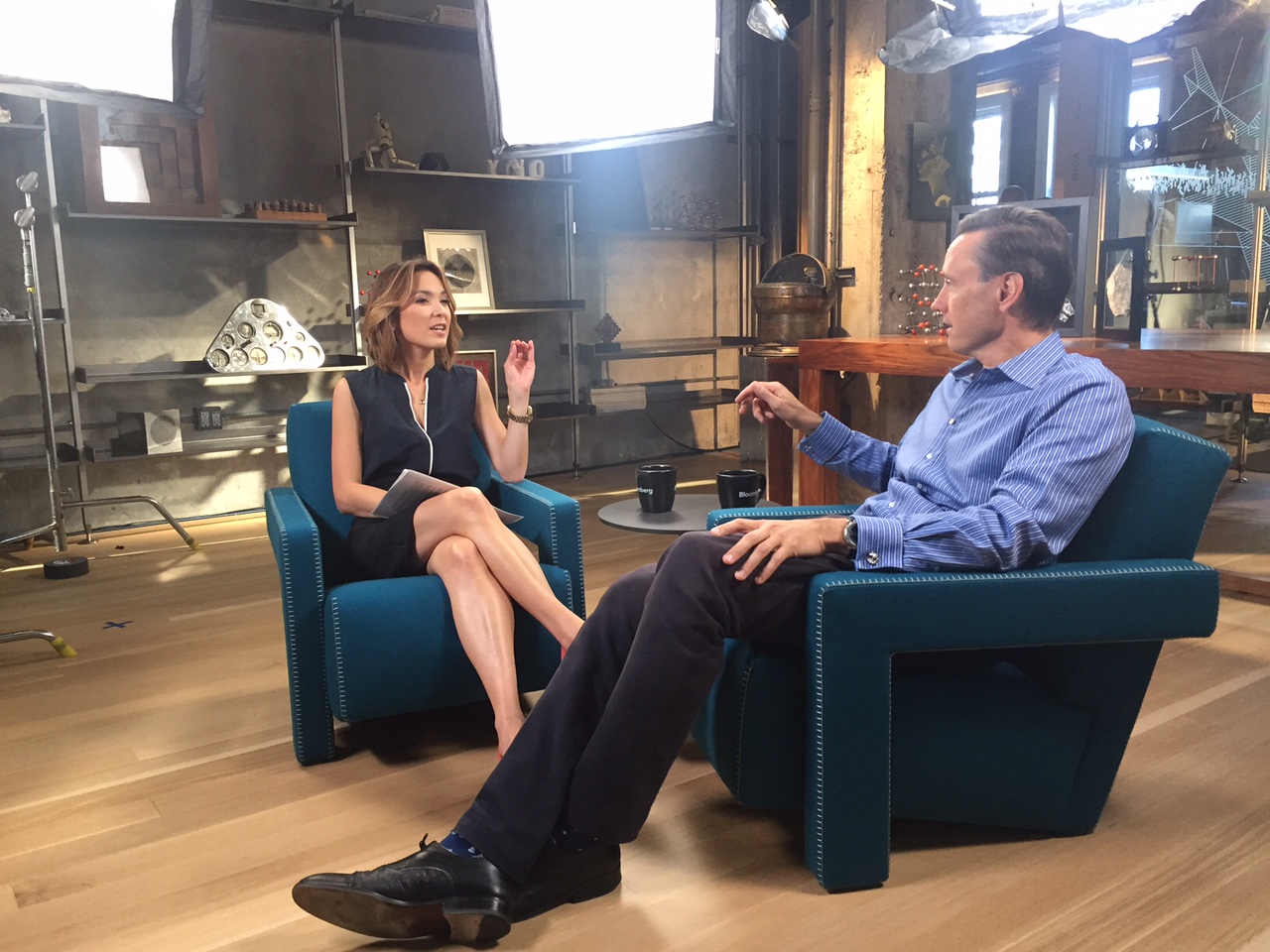
A review of Brotopia: Breaking Up the Boys’ Club of Silicon Valley by Emily Chang. Portfolio (February 2018) 320 pages.
Brotopia: Breaking up the Boy’s Club of Silicon Valley by Emily Chang subjects the software business to vigorous criticism. The dust jacket claims Silicon Valley is a “Brotopia” where “men hold all the cards and make all the rules.” Women are “vastly outnumbered” and face “toxic workplaces rife with discrimination and sexual harassment” where apparently “investors take meetings in hot tubs and network at sex parties.” Her call to action is to break up the “boy’s club” and establish gender parity in software.
Alas, such argument as the book offers is colourful mud-slinging on the basis of anecdotes. The book does not support its claims with any statistical or causal analysis. When such analysis is done Chang’s claims can be dismissed as lurid gossip based on harmful stereotypes.
Employment Complaint Statistics in California
Every year the Department of Fair Employment and Housing (DFEH) publishes an annual report that gives figures on employment and housing complaints within the State of California. Figures are given by county. I examined the annual report over the last four years to see if Silicon Valley (Santa Clara County) was a “hot spot” for complaints. I took the figures for each county over the past four years (2014-2017) and divided them by the population of the county as reported in the 2017 US Census estimate. This gives a figure for complaints per capita per annum. The results are in Table 1.
The first row is Santa Clara County. The next eight make up the other counties of the San Francisco Bay Area. Then I provide a total for the Bay Area followed by data on Los Angeles and a few other large counties in California.
County | 2017 Population (‘000s) | Average Complaints Per Year (2014-2017) | Complaints Per Year Per Million Inhabitants |
Santa Clara | 1,938 | 514 | 265 |
Alameda | 1,663 | 731 | 440 |
San Francisco | 884 | 555 | 628 |
Contra Costa | 1,147 | 413 | 360 |
San Mateo | 771 | 281 | 364 |
Solano | 445 | 176 | 396 |
Sonoma | 504 | 154 | 306 |
Marin | 261 | 109 | 418 |
Napa | 141 | 51 | 362 |
Total San Francisco Bay | 7,754 | 2719 | 351 |
Los Angeles County | 10,164 | 6314 | 621 |
Orange County | 3,190 | 1428 | 448 |
San Diego | 3,338 | 1125 | 337 |
Sacramento | 1,531 | 886 | 579 |
San Bernadino | 2,157 | 827 | 383 |
Table 1: DFEH complaints per million inhabitants
Santa Clara County contains San Jose and Palo Alto, the main centres of Silicon Valley. It is where you will find Stanford University, the head offices of Apple, Google and firms spawned by the “Paypal Mafia” (Paypal, Tesla, LinkedIn, Palantir) whose “meritocracy” is much maligned by Chang. As the data indicates, there is no indication that employment and housing complaints are particularly heavy there. On the contrary the data shows that Silicon Valley generates a complaint rate that is relatively low.
Complaints regarding sexual harassment and discrimination make up around 15 percent of DFEH complaints. The other 85 percent relate to housing and discrimination on the basis of factors other than sex such as race, disability, and so on. On the face of it, there is no obvious support in the data for Chang’s suggestion that Silicon Valley is some kind of “black spot” generating far more than its fair share of complaints due to being filled with “toxic workplaces rife with discrimination and sexual harassment.” When you apply the figure of 15 percent to 265 you get 40 complaints per year. This is 40 complaints too many, of course. Ideally there should be no complaints. One can also plausibly argue that sexual discrimination and harassment are under-reported. However, the point I am making here is not that there is no sexual discrimination or harassment in Silicon Valley. The point is simply that the complaints data does not suggest that Silicon Valley is “rife” with sexual discrimination and harassment or worse than California more generally. On the contrary, Silicon Valley seems better than average.
It is worth noting that the complaints data is categorized by the county of residence of the complainant. So, for example, a coder who lived in Napa but worked at Google or Apple and made a complaint would appear in the Napa statistics not the Santa Clara statistics. Even so, when one compares the whole San Francisco Bay Area to other metropolitan areas such as Los Angeles, Orange County and Sacramento, the complaint rate is still relatively low.
There is also a federal agency, the Equal Employment Opportunity Commission (EEOC) that handles complaints. If applicable, complaints made in California are dual-filed with both federal (EEOC) and state (DFEH) agencies under work-sharing agreements. However the reports available on the EEOC website do not provide a county-level breakdown, only a state-level one. According to the EEOC website, 373 charges for sexual harassment were filed in California in 2017. When one divides the number of EEOC complaints regarding sexual harassment by state population, California ranks 36 out of the 50 US states. The top five states for sexual harassment on a per capita basis in 2017 were Florida, Mississippi, Alabama, Georgia and Arkansas.
Female Refugees from Software
Chang argues that the “boy’s club” of Silicon Valley needs to be broken up. She paints a picture of what you might term “female software refugees” using some vivid anecdotes. The underlying themes are that a stereotype of software aptitude was created to exclude women, women do not get enough credit, women are passed over for promotion, women are not taken seriously, women start-up founders are not backed by venture capitalists. Overall, Chang claims, women are driven out of software or denied opportunity by male discrimination and harassment.
Chang does not consider any alternative theses that might cause gender disparities in occupational sectors.
For example, to explain why relatively few men nurse and relatively few women code it is not, in my view, necessary to postulate the hypotheses of patriarchy (systematic male oppression of women) and sexism (systematic male discrimination against women) as exclusive, main or even significant causes of low female participation in software. One can turn to traits well-known to psychology documented in books published over a decade ago such as Susan Pinker’s The Sexual Paradox and Simon Baron-Cohen’s The Essential Difference. People are free to choose their occupation based on their skills and their interests. It so happens relatively few women like coding and relatively few men like nursing. It is not that women can’t code and men can’t nurse. It is just that they choose to do other things.
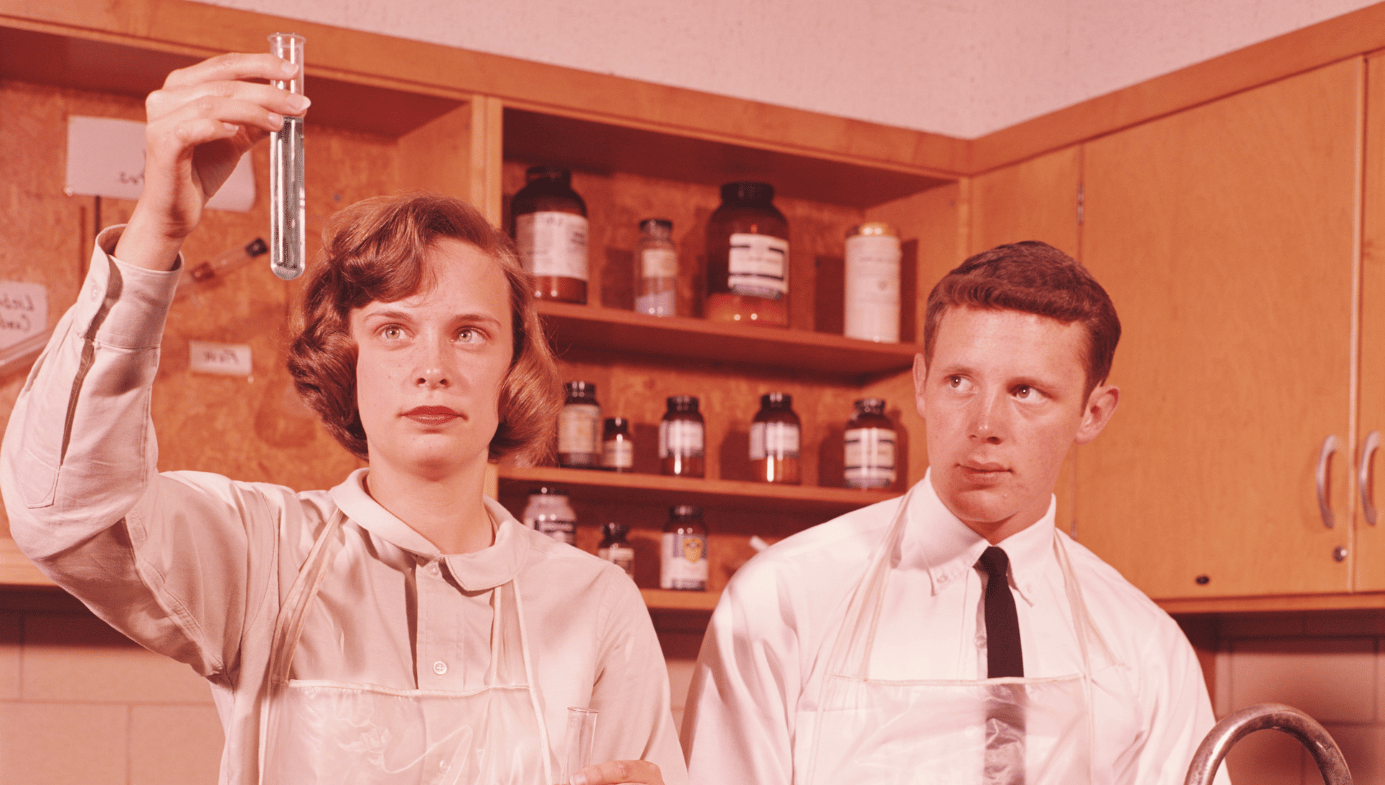
Gender Parity Statistics in the United States
Chang’s “fix” to the problem of female software refugees who are put off entering the “pipeline” of software or who quit the “leaky bucket” prematurely due to male malevolence is to break up the “boy’s club” of Silicon Valley. Alas, she provides no plan as to how this major workforce change will be achieved. Nor does she offer any justification as to why the “boy’s club” of software engineering should be broken up while “girl’s clubs” like nursing, elementary school education, psychology, and human resources management should be left as they are. Most importantly, Chang shows no awareness that gender parity is the exception not the rule in the US workplace. She assumes that gender parity in software engineering is right and should be implemented. She argues that lack of parity in software is due to male malevolence, exclusion, and bias.
I define ‘parity’ and related notions such as ‘female majority’ and ‘male dominant’ to categorize gender equality (or the lack of it) in occupational sectors as shown in Table 2.
Classification | Women % |
Male dominant | 0-19.99 |
Male majority | 20-39.99 |
Parity | 40-59.99 |
Female majority | 60-79.99 |
Female dominant | 80-100 |
Insufficient data | ? |
Table 2: Gender equality classifications used in this article
The US Department of Labor provides a report which lists occupational sectors and gives a percentage of the women working in each sector that has more than fifty thousand workers. The 2017 report lists 535 occupational sectors. These are arranged into five overarching categories as shown in Table 3.
Occupation | Total employed (000s) | Women % | Classification |
Management, professional, and related occupations | 60,901 | 51.6 | Parity |
Service occupations | 26,751 | 56.6 | Parity |
Sales and office occupations | 33,566 | 61.3 | Female majority |
Natural resources, construction, and maintenance occupations | 14,193 | 5.1 | Male dominant |
Production, transportation, and material moving occupations | 17,927 | 22.8 | Male majority |
Total, 16 years and over | 153,337 | 46.9 | Parity |
Table 3: US Labor Department employment figures by gender
When one drills into the 535 occupational sectors and applies the classifications in Table 3 we arrive at Table 4.
Classification of occupational sectors by gender equality | Sectors | Workers (millions) |
Female dominant | 42 | 25.6 |
Female majority | 68 | 28.7 |
Parity | 72 | 29.7 |
Male majority | 57 | 32.6 |
Male dominant | 92 | 32.8 |
Total sectors where female participation is recorded | 331 | 149.4 |
Insufficient data | 204 | 3.9 |
Total | 535 | 153.3 |
Table 4: Occupational sectors by gender equality classifications
Of the 331 occupational sectors, having at least 50,000 workers in them, where female participation is recorded, only 72 (21.8 percent) have parity. 78.2 percent do not.
Looking at the numbers of workers, rather than the number of sectors, we find that the labour force in sectors with parity makes up 19.9 percent of the total where female participation figures are given.
Either way, in round numbers, only 1 in 5 people in America works in an occupational sector that has gender parity.
Parity, in short, is the exception not the rule.
Root Causes of Gender Disparity
This leads to various questions. What are the root causes of gender disparity in occupational sectors in general? What, if anything, should be done about gender disparity? What causes disparity in software in particular? What, if anything, should be done about gender disparity among software developers and computer programmers who collectively are only 19.3 percent female? What, if anything, should be done about gender disparity in psychology (68.9 percent), nursing (89.9 percent) and preschool and kindergarten education (97.7 percent)?
It has to be said, if there is a chronic bias in hiring it is happening on the watch of a female majority occupational sector. Human resources (HR) is a “girl’s club” at both management (70.8 percent) and worker levels (72.1 percent).
Answers to the general questions can be found in the psychology literature (e.g. Pinker and Baron-Cohen). As for the more specific software questions, I would say writing software suits systemizing, deep introverts with high intelligence, single-mindedness, grit, tenacity and a degree of obsession. You can survive in software with less empathy than a retail sales clerk in a clothing store. Provided that you are conscientious and can code, you can get away with a high degree of “mindblindness” and a lack of social graces. If you can write bug-free algebraic code that compiles and meets requirements, your personal quirks and inadequacies can be worked around. Po Bronson’s wonderful The Nudist on the Late Shift provides some colourful examples of the psychological idiosyncrasies found at the extremes of the talent distribution among coders. Such traits are more common in males. The root causes are 1) fetal testosterone and all that goes with it in terms of trait distributions between the sexes; 2) cultural factors that amplify sex differences; and, 3) free choice of occupation.
It is far from clear to me that software has a problem. Even if you assume that gender disparity in occupational sectors is bad and is not the natural result of men and women making choices based on their talents, aptitudes and interests, software is far from being the worst offender.
Most other branches of engineering employ fewer women than software. Civil (14.4 percent), mechanical (9.2 percent), electrical (12.3 percent), chemical (16.9 percent) and aerospace engineering (8.9 percent) all have lower rates of female participation than software (19.3 percent). Indeed, some areas related to software engineering achieve gender parity as I have defined it. For example, database administrators are 40.6 percent female. Others do reasonably well. For example, there are more female web developers (32.3 percent) than there are male psychologists (31.1 percent) and 38.9 of computer systems analysts are female. Software runs rings around carpentry (2.2 percent) and plumbing (2.2 percent).
The top five occupational sectors in terms of male domination are: Roofers (0.6 percent); electrical power line installers and repairers (0.6 percent); brick masons, block masons, and stone masons (0.7 percent); cement masons, concrete finishers, and terrazzo workers (1.0 percent); and automotive body and related repairers (1.1 percent).
Writing software is not even in the top 50 occupational sectors ranked by male dominance. For some reason (probably historic), the US Labor Department distinguishes between “computer programmers” (21.2 percent) and “software developers, applications, and systems software” (18.7 percent). Merging these two fields, I get an aggregate of 19.3 percent. Software sits alongside other male dominated fields such as dishwashers (19.5 percent), couriers and messengers (19.4 percent) and taxi drivers and chauffeurs (18.9 percent). Chang says nothing about breaking up those “boy’s clubs.”
In terms of female domination the top five sectors are: Speech-language pathologists (98.0 percent); preschool and kindergarten teachers (97.7 percent); dental assistants (95.9 percent); secretaries and administrative assistants (95.0 percent) and dental hygienists (94.9 percent).
The five most evenly balanced sectors are counter and rental clerks (48.9 percent), lifeguards and all other protective service workers (49.6 percent), optometrists (50.2 percent), “sales and related workers, all other” (50.3 percent), and advertising sales agents (50.4 percent).
Gender disparity is far from unusual, occurring in four out of five occupational sectors. Chang makes no complaint about gender disparity in female dominant fields. However, if the goal of achieving gender parity in software is to be attained, then we must tip some of the men out of software and tip some women in. On the 2017 data, the number of those classified by the US Labor Department as computer programmers and software engineers is two million. Of these, only 388,000 are female. To achieve parity at the 2017 level of demand, we would need to find 622,000 women to move into coding and, by the same token, we would need to move 622,000 men out. If this is somehow done, then there will be gender parity and, one hopes, all will be well in the tech world.
Where are these women going to come from? As a thought experiment, we could dump them out of “girl’s clubs” like kindergarten teaching and speech-language pathology, though given the large numbers we need, we would have to raid the big battalions of female employment: nursing and teaching. But, as an exercise of the imagination, we could move 622,000 women out of the 2.7 million women currently in nursing and replace them in hospitals with 622,000 male software engineers.
Is this going to improve the world? I think not. There is no evidence that empathetic multi-tasking nurses really want to code. Nor is there any evidence that systemizing monotasking coders really want to nurse.
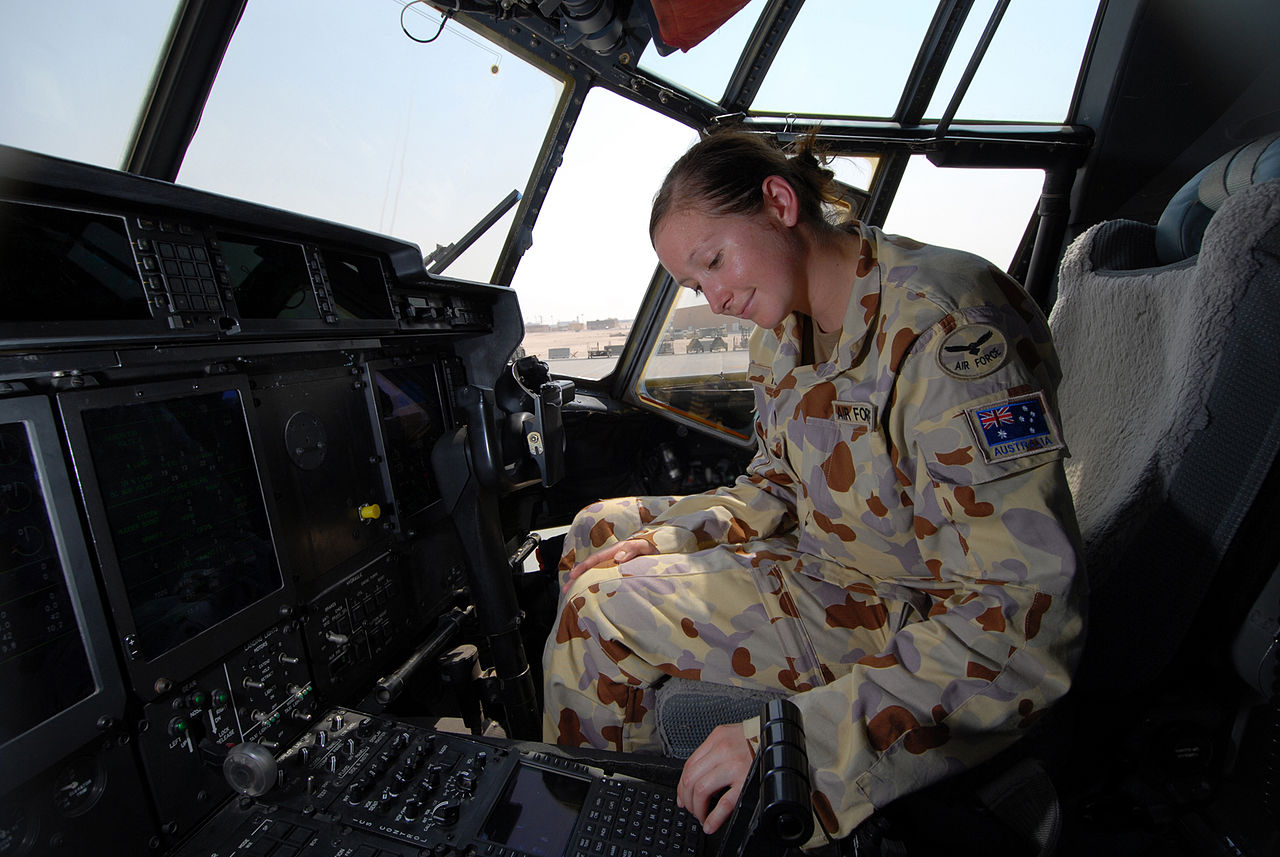
Chang does argue that women are put off going into coding. I don’t doubt this is true. However, much the same problems exist for men going into nursing. Personally, I never gave nursing any serious consideration as a career even though my mother was a nurse. Men in nursing complain about their teachers all being female, feeling like fish out of water, having colleagues who do not share their interests and not being taken seriously. Many stories told by male nurses are much the same as those told by female coders in Brotopia.
It is possible to get gender parity in nursing. The Iranians achieved it for a while during the Iran-Iraq War in the 1980s. However, as soon as the emergency of war was over, the male recruits dropped out of nursing in droves. Similarly, it is possible to get to parity in engineering. The Soviet Union simply told people what they could study. By restricting choice, you can get to parity easily. In jurisdictions where there is little choice, there are lots more female engineers (e.g. India). However, once there is a wider range of choice, women choose other occupations.
On the US data, there are 535 occupational sectors to choose from. Given the range of choice and the distribution of traits that are likely to influence aptitude, I do not see any compelling reason why we should be perturbed if fewer women choose to code than men. Nor should we worry that fewer men choose to nurse than women.
Speaking for myself, I have no problem with women in STEM in general and women in software in particular. If you are female and seeking to found a “unicorn” don’t waste time reading Chang’s jealous whines about the runaway successes of the close-knit Paypal Mafia. Read From Zero to One by the “Don” himself, Peter Thiel. Then pick a close-knit team with skills diversity and clear commercial purpose yourself.
To conclude, what motivates Brotopia is gossip and envy. It is an opportunistic ride on a mythical wave. Chang ignores psychology findings that do not fit her narrative theme of female suffering at the hands of men. She avoids discussing well-known results, some published over a decade ago, that can provide far less sinister explanations as to why more men don’t nurse and more women don’t code.
On the DFEH data, Silicon Valley has no obvious case to answer. If anything, it deserves a pat on the back for having just over half the complaint rate of Los Angeles. If feminists wish to persuade men who work with data and numbers all day every day that there is something seriously wrong with their industry, they need to find hard data and crunch the numbers.
There is no excuse not to: 48.8 percent of US statisticians are female.