Noise: A Flaw in Human Judgment—A Review
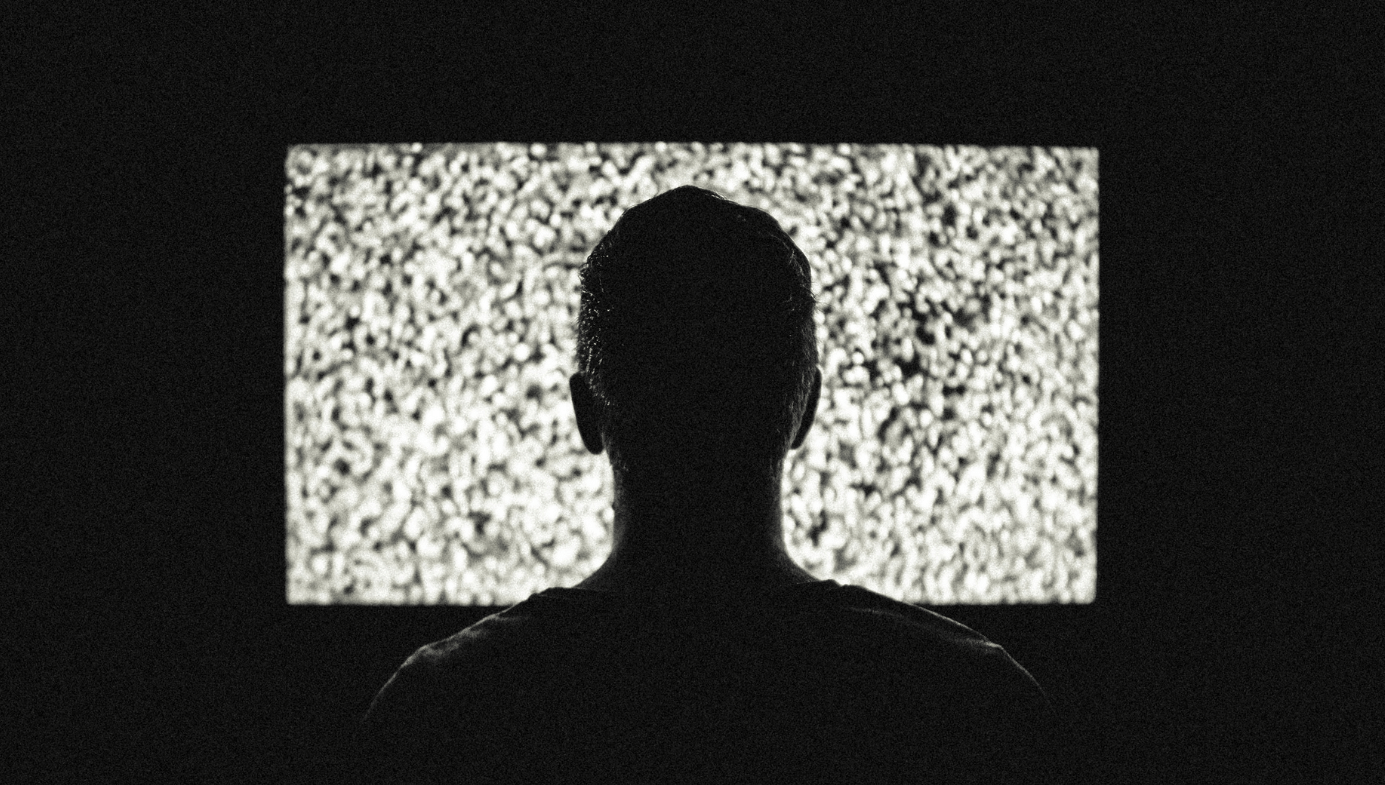
A review of Noise: A Flaw in Human Judgment by Daniel Kahneman, Oliver Sibony, and Cass R. Sunstein. Little, Brown and Company, 464 pages (May 2021)
Are crowds smart or dumb? You may have heard the terms “wisdom of the crowds” and the “madness of crowds.” The former idea is that the collective opinion of a group of people is often more accurate than any individual person, and that gathering input from many individuals averages out the errors of each person and produces a more accurate answer. In contrast, the “madness of crowds” captures the idea that, relative to a single individual, large numbers of people are more likely to indulge their passions and get carried away by impulsive or destructive behaviors. So, which concept more accurately reflects reality?
Noise: A Flaw in Human Judgement by Daniel Kahneman, Olivier Sibony, and Cass R. Sunstein provides the answer. The authors share research indicating that “independence is a prerequisite for the wisdom of crowds.” That is, if you want to use crowdsourcing to produce accurate information, you have to ensure that people make their judgments in private. If people provide their answers in a public setting where they can see everyone else’s answers, then the crowd can transform wisdom into madness.
Relatedly, Wharton organizational psychologist Adam Grant has stated that “you get more and better ideas if people are working alone in separate rooms than if they are brainstorming in a group. When people generate ideas together, many of the best ones never get shared. Some members dominate the conversation, others hold back to avoid looking foolish, and the whole group tends to conform to the majority’s taste.” People converge on ideas they believe are held by the majority, when often they are simply acquiescing to the most assertive and strident members of the group. Grant suggests that the best way to sidestep this problem is to have people think up ideas on their own before the group evaluates them.
In Noise, Kahneman and his colleagues report findings indicating that, in tasks involving estimation, such as the number of crimes in a city or geographic distances, crowds are wise as long as they registered their views independently. But if people learn what others estimated, then crowds do worse than individuals. The book states that, “while multiple independent opinions, properly aggregated, can be strikingly accurate, even a little social influence can produce a kind of herding that undermines the wisdom of the crowds.”
There are many reasons why Kahneman’s 2011 book Thinking, Fast and Slow became a groundbreaking hit, while Noise did not reach quite the same heights (Kahneman and his statistically savvy co-authors might cite regression toward the mean). One reason for the difference may be the years in which the books were published. In 2011, the educated class generally favored meritocratic and objective measures for judgment and decision-making. They found the message that we should challenge the role of bias in our everyday judgments appealing, and believed that we should rid ourselves of habits that lead us to judge other people or situations unfairly. Today, however, much of intellectual culture has changed. Now that luxury beliefs are ascendent, relying on objective measures is no longer fashionable.
With the paperback version of Noise slated to come out in May 2022, it is worth delving into some of the key arguments contained within this book. It is filled with fascinating insights relevant to current social and political debates.
The book begins by making a distinction between two kinds of error. The first—bias—is well-known, in no small part thanks to Kahneman’s influence. The second—noise—is less well understood. Bias refers to judgments that depart from rationality in consistent, predictable ways. If you ask people to estimate the likelihood of dying in a plane crash, you can predict their answers will generally be biased upward because airline accidents are so vividly covered in the media and readily come to mind (the availability bias). In contrast, noise means that judgments are unpredictable, or scattered. If you ask a group of people to guess the weight of a bull, their answers will not be biased. They will be noisy, meaning they all depart from the true weight of the bull in unforeseeable ways.
Bias is characterized by systematic deviation in a predictable manner. Noise refers to random scatter. Interestingly, you don’t need to know the true answer to a question in order to infer the presence of noise in people’s judgments. For instance, if you ask 10 different physicians to diagnose a patient and they each return a different answer, then you can conclude that their answers are noisy, even if the true diagnosis remains unknown.
Noise is readily apparent in legal decisions. The authors point out that judges exercise a great deal of discretion when determining appropriate sentencing. Many consider this both just and humane, believing that criminal sentences should be tailored to the defendant’s characteristics and circumstances. And yet Kahneman and his colleagues report research from the 1970s and 1980s, indicating that sentencing depended less on the specific case or the individual defendant than on the individual judge. That is, the same defendant in the same case might get wildly different sentences depending on which judge is reviewing the case. One study found that a heroin dealer could be incarcerated for between one and 10 years, depending on the judge. In another study on burglary, the recommended sentences ranged from five years in prison to a mere 30 days. In a larger study involving 208 US federal judges who evaluated the same 16 hypothetical cases, in only three of the 16 cases was there unanimous agreement to impose a prison term.
In 1984, the US Congress enacted the Sentencing Reform Act in order to reduce noise in the legal system by reducing what the book describes as “the unfettered discretion the law confers on those judges and parole authorities responsible for imposing and implementing the sentences.” And yet, although the difference in sentence length between similar cases fell dramatically after the introduction of the guidelines, critics argued that these new guidelines were unfair because they prohibited judges from taking adequate account of the particulars of the case. “The price of reducing noise,” the authors write, “was to make decisions unacceptably mechanical.” Some people are deeply uncomfortable with the elimination of noise, perhaps because they like the idea of appealing to subjective human discretion. Suppose you were a defendant and had to choose between two scenarios. In one, if you are found guilty you are guaranteed to serve three years. In the other, if you are found guilty you will serve either 30 days or 10 years (or something in between). Many would favor the latter.
In another study on underwriters and insurance claims adjusters, the authors asked 828 CEOs and senior executives to guess how much variation they expected to find in judgments of insurance premiums. They guessed 10 percent or less. But the median difference in underwriter’s judgments was 55 percent. The book states that for insurance claims, “the price a customer is asked to pay depends to an uncomfortable extent on the lottery that picks the employee who will deal with that transaction.” Likewise, case officers in child protective services vary in how likely they are to place children in foster care.
Of course, noise in some judgments is desirable, such as in matters of preference or taste. If 10 film critics watch the same movie, or 10 people read the same novel, you’d both expect and welcome a variety of opinions. But noise is both undesirable and unfair when it comes to medical diagnoses, criminal sentencing, insurance claims, employee selection, and college admissions, among other decision-making domains.
What are the sources of noise? One worth highlighting—because it may be the most pernicious—is simply the discomfort of disagreement. Most organizations prefer consensus and harmony over dissent and conflict. Indeed, the book points out that “The procedures in place often seem expressly designed to minimize the frequency of exposure to actual disagreements and, when such disagreements happen, to explain them away.”
Relevant to current debates about college admissions, Noise contains a story from a university professor who was helping his admissions office review its decision process. He explained their process for selecting applicants. First, a person read an application file, rated it, and then handed it off with ratings to a second reader, who also rated it. As you can imagine, the first rater holds much more sway than the second, who might be reluctant to challenge the initial evaluation. The professor suggested masking the first reader’s ratings so as not to influence the second reader.
In other words, he suggested they use the “wisdom of crowds” method rather than the “madness of crowds” approach. The school’s reply: “We used to do that, but it resulted in so many disagreements that we switched to the current system.” As Kahneman and his colleagues point out, many organizations consider conflict avoidance to be at least as important as optimal decision-making.
As more and more top universities are electing to ditch standardized tests, they will be relying on noisier forms of evaluation. Removing information when making decisions is generally unwise. However, removing parts of an application that contain more objective information—test scores as opposed to softer metrics such as essays—will likely inflate the amount of noise to an even greater degree.
In fact, recent research from Stanford found that family income is more highly correlated with admissions essay content than with SAT scores. Presumably, applicants from well-to-do backgrounds are especially adept at crafting their essays in ways that please admissions committees. On the topic of standardized testing, Kahneman and his colleagues report that “Intelligence is correlated with good performance in virtually all domains. All things being equal, it is associated not only with higher academic achievement but also with higher job performance” and it “remains by far the best single predictor of important outcomes.”
In Noise, Kahneman and his co-authors take pains to note that good decision-making should not mix facts and values. Instead, good decision-making must be based on accurate predictive judgments that are not affected by preferences or values.
The book presents persuasive evidence of “level noise,” or the variability in the judgments produced by different people. Level noise encompasses the idiosyncratic responses from each unique person making a decision.
Perhaps more interesting, though, is what the book describes as occasion noise, or the variability in the judgments produced by the same person. In other words, occasion noise involves your own idiosyncratic judgments that might be affected by mood, weather, time, and so on. For example, how you rate the quality of an essay first thing in the morning after you’ve had your coffee might be different from how you rate it late at night after a stressful day at work.
Consider recent research out of Cambridge University on credit loan applications. Tobias Baer and Simone Schnall examined the decisions of credit officers at a major bank. They found that the officers were significantly more likely to grant loan repayment terms to a customer in the morning compared with later in the day, when decision fatigue impaired their ability to carefully assess applications, and so they tended to default to “no.”
The book reports research indicating that when the same software developers were asked on two separate days to estimate the completion time for the same task, their answers, on average, differed by 71 percent. It is also common to obtain significantly different diagnoses from the same physicians when they are presented with the same case twice. A study of nearly 700,000 primary care visits found that physicians are significantly more likely to prescribe opioids and antibiotics at the end of a long day. It seems that when doctors are tired and under time pressure, they are more inclined to choose a quick-fix solution.
But occasion noise doesn’t just affect doctors. When wine experts at a prominent wine competition tasted the same wines twice, they only scored 18 percent of the wines identically—usually, the very worst ones. This suggests that while people tend to agree with themselves on which wines are bad, they often disagree with themselves on which ones are good.
I have wondered about occasion noise in my own judgments. For instance, when asked to provide comments on another person’s writing, there are times when I question whether my feedback would have been different on a different day of the week, before or after lunch, and so on. For my own writing, I often send important drafts to a few different people, seeking what psychologists describe as “inter-rater reliability.” I will typically only implement large changes if two or more people make the same suggestion for improvement.
Kahneman and his co-authors are blunt in their conclusion: “We do not always produce identical judgments when faced with the same facts on two occasions … you are not the same person at all times.” That is, as your mood and external circumstances vary, some features of your cognitive machinery vary too.
In short, you are a source of a lot of noise. While some degree of occasion noise is due to weather, stress, fatigue, and so on, much of it may simply be normal. The book describes studies in which researchers administered cognitive tests to participants at different periods. They found that various external factors including time of day, sleep, and so on accounted for only 11 percent of the variance in the performance of a given person. The authors interpret these findings as evidence that the moment-to-moment variability in brain functioning is driven not just by external influences, but is instead characteristic of the way the brain functions.
Somewhat reassuringly, at least, occasion noise is smaller than level noise. In other words, you agree with your own judgments less often than you think. But you still tend to agree with yourself more often than with others.
Noise can be amplified by groups. For example, researchers carried out an experiment on a website that allows viewers to comment on stories and vote on different comments. You might think that a single initial vote on a comment would not influence the long-term popularity of the comment, but you’d be wrong. When people see an initial up vote on a comment, they are 32 percent more likely to also give it an up vote. A slight boost in the initial popularity of a comment led to an artificially increased mean rating by 25 percent after five months.
Initial starting points can influence political views too. The book reports findings indicating that when a group of Democrats saw that a particular view was gaining initial popularity among Democrats, they too would endorse that point of view, ultimately leading most Democrats in the group to support it. However, if they saw that a specific opinion was gaining popularity among Republicans, they rejected it. Republicans behaved similarly. In short, the acceptance of a viewpoint can depend on its initial popularity and the specific group that accepts it.
This relates to another topic within the book: Group polarization. This is a special case of the “madness of crowds” phenomenon. Social psychologists have found that when individuals hold certain beliefs, they become more extreme in their beliefs when they interact with others who hold similar views. In a study on jury behavior, researchers gave jurors an eight-point scale to measure how severely they wanted to punish a law-breaker. They found that when individual jurists preferred severe punishment, the overall verdict ended up higher than that recommended by the median juror.
Put differently, when individual jurors preferred a severe punishment, deliberation with other jurors sharing this view raised the overall severity of the punishment. One juror might say they want to impose a fine of $10,000, while another might say that anything less than $12,000 is unacceptable. By the end, the fine might increase to an amount far beyond anyone’s initial starting point. Conversely, groups comprised of lenient jurors produced even more lenient verdicts than the one recommended by the median juror in the group. When group members drift in a certain direction, individual members will double-down on that perspective. This drives the group towards extremism even though individual members are not extreme.
So what is the best way to make decisions?
The book distinguishes between clinical judgments and models. For clinical judgment, you consider the information at hand, perhaps engage in a quick mental computation, consult your intuition, and come up with a judgment. Suppose you are evaluating two candidates. You engage in some deliberate reasoning, compare their resumes, references, and interview performances. This process, plus your gut feeling, is what leads to your clinical judgment.
In contrast, the book describes simple models. This comes from work by the mid-20th century psychologist Paul Meehl. He pitted clinical judgment against mechanical prediction for outcomes such as academic success and mental health prognoses. Meehl, a practicing psychoanalyst, was surprised to discover that simple mechanical rules were generally superior to human judgment.
Relatedly, in a separate line of research, Lewis Goldberg and colleagues built models of human judges. They had people make a variety of judgments, input their responses into a computer, then they built simple models intended to replicate these people’s judgments. The judgments of the models were highly correlated with the judgments of the humans they were intended to imitate (r =.80). Crucially, though, for real-world outcomes, the models were more accurate than the people. For instance, the models and the people had to predict the GPAs of 98 students using 10 simple inputs. For every one of the 98 students, the model did better than the actual humans. They found similar results for other kinds of judgments. A crude model of your judgments is likely to be more accurate than you are. The book’s authors conclude that “Complexity and richness do not generally lead to more accurate predictions.” In other words, using straightforward models with simple inputs is superior to “holistic” judgments for predicting real-world outcomes.
But why is this the case? It seems that the very things we most cherish in humans may lead our judgments astray. Replacing you with a model of you eliminates your subtlety, and eliminates your noise. If I present you with the same candidate at two different periods, you may have very different judgments. But if I present a model of you with the same candidates, it will produce identical judgments both times. As Kahneman and his colleagues put it, “You may believe that you are subtler, more insightful, and more nuanced than a linear caricature of your thinking. But in fact, you are mostly noisier … it proved almost impossible in that study to generate a simple model that did worse than the experts did.”
A human’s informed judgment, often guided by less-than-rational gut feelings, typically performs worse than simple statistical formulas. In another example, researchers used two variables to predict a defendant’s likelihood to jump bail: age (older people are lower flight risks) and number of past court dates missed (people who have failed to appear before are likelier to recidivate). This simple model outperformed predicted flight risk better than all human bail judges.
Why do we often favor subjective human judgments over straightforward rules?
Many experts ignore the clinical-versus-mechanical debate and prefer to trust their own judgment instead. The use of algorithms is not yet routine in medical diagnoses. Few businesses or universities use algorithms when selecting candidates. Hollywood executives green-light movies based on their judgment and experience rather than formulas, and book publishers do the same.
When experts listen to their gut, they experience emotionally satisfying rewards, especially when they are proved “right” in the end (overlooking or dismissing all the times they were wrong). Giving up such rewards in favor of more accurate but less intuitively satisfying models is not easy. As Kahneman and his co-authors state, “Many decision makers will reject decision-making approaches that deprive them of the ability to exercise their intuition.”
Interestingly, though, when given the choice, ordinary people often prefer advice from an algorithm rather than a human. They’ll give the algorithm a chance, but will stop trusting it as soon as they see it make a mistake. The book states that “As humans, we are keenly aware that we make mistakes, but that is a privilege we are not prepared to share. We expect machines to be perfect.”
This explains why so many people do not trust self-driving cars. If a human driver causes a traffic collision, we are often forgiving, depending on the circumstances. But if an autonomous vehicle causes an accident, people respond with intense suspicion. To borrow a popular phrase from Charlotte Whitton, a robot must do twice as well as a human to be thought half as good.
Relatedly, recent research led by NYU professor Andrea Bonezzi suggests that the reason people tend to trust humans more than algorithms is that while people see humans as discernible decision-makers, we typically view computers as a “black box.” We foster an illusion that we understand how others come to arrive at their decisions because we project our own intuitive understanding of decision-making onto them. However, we are often mistaken in how other people form their judgments, and we don’t project our decision-making process onto computers, which seem alien to us.
The book does offer some solutions to the problem of noise. For example, when rating job candidates, a better predictor of future performance is to rank them from best to worst rather than evaluating each one individually. As the book puts it, “replacing absolute judgments with relative ones, when feasible, is likely to reduce noise.”
Another helpful approach: If multiple examiners are called upon to assess a candidate or individual’s performance, they should provide their judgments privately—none of them should be aware of the others’ evaluations. This capitalizes on the wisdom of crowds rather than the madness of crowds.
Furthermore, noise can be reduced by using standardized ratings in a forced ranking system. In this approach, raters must abide by a predetermined distribution, which prevents ratings inflation. For example, when I was in the US Air Force, annual performance was evaluated on a scale from one to five. For a time, the majority of members were given fives, and it was considered a career death-blow to get a four, which would impede promotion to the next rank. In 2015, the Air Force revamped their system so that only a small number of people in a given unit could obtain high scores, while the rest would fill out the rest of the distribution.
Still, there are many reasons why raters might not want to be honest about a person’s true performance. They might assess people “strategically” in order to avoid a difficult discussion, to favor a person who they believe deserves a long-awaited promotion, or to get rid of an underperforming employee who needs a good evaluation to be transferred to another division. Such hidden motives can introduce noise into important decisions.
And this, I suspect, is the reason why some people may not like the book. They want to retain noise (the ability to rely on intuition and individual judgment), and therefore they will dislike Noise (which argues that relying on intuition is harmful and unfair). Any organization that inhibits the noise attributable to human judgment reduces people’s ability to influence ratings in pursuit of their own goals.
If you tell people they can no longer rely on their gut feelings and that they must follow a checklist or abide by an algorithm, they will respond with resistance because such policies inhibit their ability to pursue their own hidden agendas. Others may argue that guidelines designed to eliminate noise are rigid, dehumanizing, and unfair. Many people have had the experience of making a seemingly reasonable request to an organization only to receive the response that clear rules prevent the organization from making any exceptions. Many people who plead for exceptions really do believe they are exceptional. But for many decision-makers, they encounter people asking for such exceptional requests all the time. The rules, as the authors put it, “may seem stupid and even cruel, but they may have been adopted for good reason: to reduce noise (and perhaps bias as well).”
When people believe an objective system favors them, they want to reduce bias and noise. And when people believe an objective system might disfavor them, they want to retain bias and noise. But Kahneman and his colleagues make a persuasive case that eliminating noise is crucial for a system to maintain legitimacy. Near the end of the book, they conclude that, “It is unfair for similarly situated people to be treated differently, and a system in which professional judgments are seen as inconsistent loses credibility.”
If they are right, then perhaps more people should carefully consider the policies they are supporting. Unfortunately, people who favor noise are often the noisiest.