Will AI Spell the End of Capitalism?
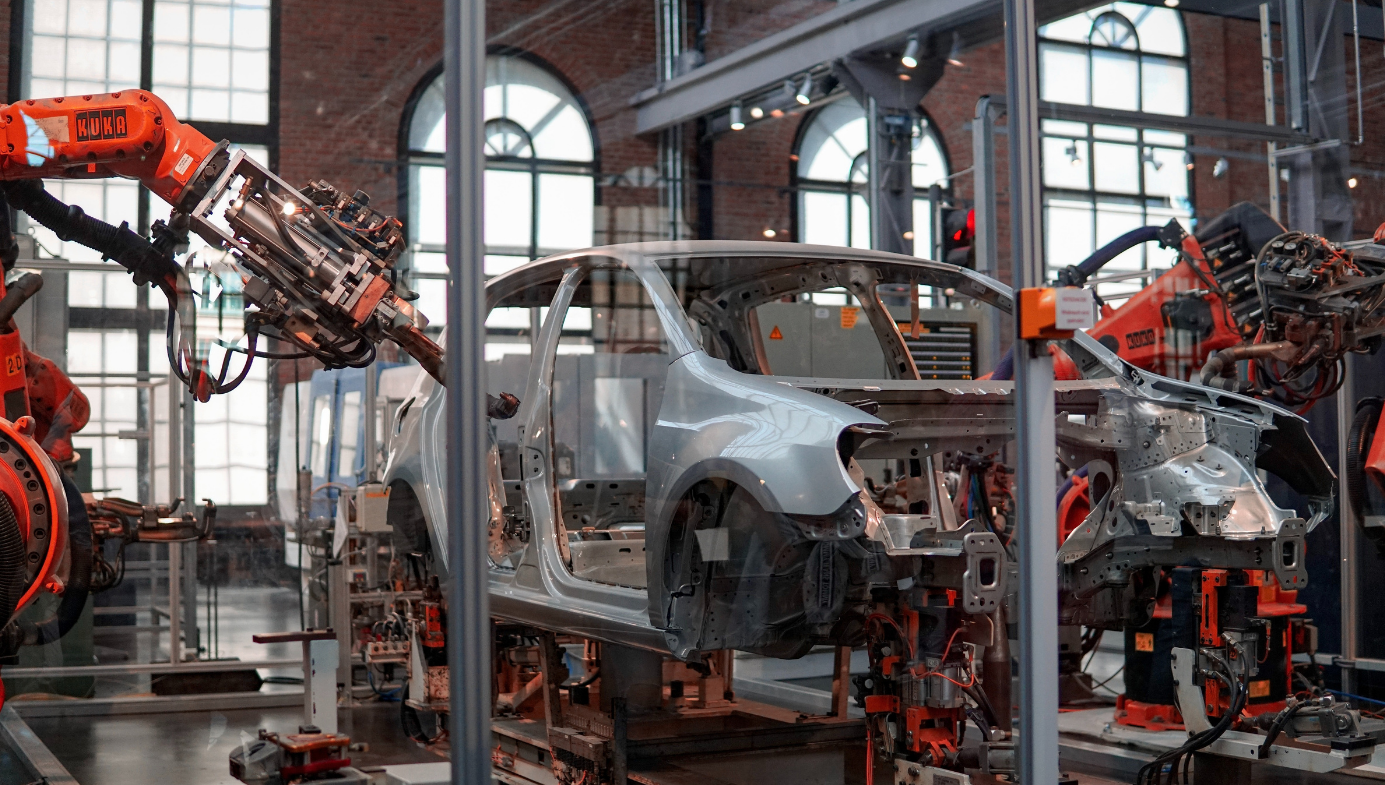
In 2018, the Washington Post published an opinion piece by Feng Xiang entitled “AI Will Spell the End of Capitalism.” Professor Feng teaches law at Tsinghua University and is one of China’s most prominent legal scholars. The core of his argument is set forth in the opening paragraphs:
If AI remains under the control of market forces, it will inexorably result in a super-rich oligopoly of data billionaires who reap the wealth created by robots that displace human labor, leaving massive unemployment in their wake.
But China’s socialist market economy could provide a solution to this. If AI rationally allocates resources through big data analysis, and if robust feedback loops can supplant the imperfections of “the invisible hand” while fairly sharing the vast wealth it creates, a planned economy that actually works could at last be achievable.
There are a couple of things wrong with this. First, there is the obvious point that Marxists have been prophesying the end of capitalism since 1848. Second, Der Spiegel has run covers prophesying massive unemployment due to robots since 1964. These predictions are yet to eventuate.
Robots will put humans out of work. Cover of Der Spiegel in 1964, 1978 and 2017.
— Lionel Page (@page_eco) November 20, 2017
ht @gduval_altereco pic.twitter.com/QjkGDohNEq
Feng hopes that state-owned AI will revive the long-dead idea of socialist central planning. He is probably wrong about this because he overestimates the capabilities of AI and what it is good for. His reference to “big data analysis” indicates that he is referring to the particular class of data-hungry machine learning (ML) models popular today. These algorithms require a lot of data because they rely on mimicry rather than understanding and independent reasoning. ML does not work like human learning. Human children do not need ten thousand tagged images to tell the difference between cats and dogs, but contemporary ML does.
Given a particular task, if the purpose is known, a human can seek to serve that purpose even if there are surprises, i.e., unanticipated changes in circumstance. The opposite is true for a digital mimic that knows nothing of the purpose it serves. This is why anything novel or out of the ordinary tends to break ML. For example, the error rate of systems looking to distinguish feral trees from native trees can increase dramatically if the live aerial photos start to differ from the photos used to train the model. Shifts in satellite orbit, the time of day pictures are taken and even modest moves in geography and vegetation can degrade model accuracy. Contemporary ML-based AI is very much a case of highly trained horses for very specific courses.
As is commonly said, artificial intelligence is brittle (but fast) whereas human intelligence is robust (but slow). If the task is to land a drone, provide song recommendations, or even predict protein folding, then mimicry can work well, given a sufficiently varied quantity of training data. If, on the other hand, rationality or the ability to provide nuanced reasoning for past decisions is required, mimicry flops. The ability to deal with the unexpected is one of the great strengths of Homo sapiens.
Feng’s claim is simply that AI oligarchs are bad and the only credible fix is a “socialist market economy” governed by a Marxist one-party state. This is a false dichotomy; our choice is not between these two extremes. We agree that AI oligarchs are an unattractive prospect. However, there are existing remedies for cartels, monopolies, and harmful AI products in the pluralist West. Targeted regulation is a better fix for capitalism’s defects than a revolution led by an alliance of workers and peasants. As a result of Frances Haugen’s testimony, many in the US Congress are looking to clip the wings of social media. The EU has led the world in regulating AI products, introducing rights to explanations, rights to be forgotten, and rights to data privacy. The Australian government has released draft legislation to expose anonymous trolls to defamation actions by removing the “platform” shield of social media and making them “publishers” accountable for the views their users post just like traditional media. The “wild west” days of the information age are over.
But Feng offers a typically Marxist “all or nothing” argument. To fix the problems of competitive capitalism, his solution is a Marxist political monopoly based on the revolutionary expropriation of the expropriators. His argument is unconvincing because it is based on a hopelessly dated caricature of capitalism. “Laissez-faire capitalism as we have known it,” he says, “can lead nowhere but to a dictatorship of AI oligarchs who gather rents because the intellectual property they own rules over the means of production.”
The obvious problem with this argument is that laissez-faire capitalism is extinct, long since abandoned in favour of regulation, anti-trust legislation, and redistribution through the welfare state. Feng overstates the market power of the AI oligarchs, most of whom make their money selling ads in a competitive market. He says nothing about the coercive power of a political monopoly, that can silence policy competition by throwing it into the gulag.
The most sinister aspect of current AI is what a one-party state can do with it. Silicon Valley has given China the technical tools to set up the world of 1984. Now the party telescreen can monitor the likes of Winston Smith 24/7. Instead of a screen on the wall, it’s the mobile phone in your pocket connected to the Internet that can be used to track you and monitor what you click on, who and what you message, and keep you and all your fellow citizens under constant surveillance for “counter-revolutionary” views. In China, the Internet and social media have evolved to be a tyrant’s dream. Comrade political officers in technology firms monitor online posts for “objectionable” material and have unlimited powers of “moderation.”
The Achilles heel of this political strategy is that it creates a culture in which people are afraid to think and speak freely. When you have to filter every word you say in case it offends the powers that be, you are strongly motivated to avoid risky creative thinking. In a society where the state can control everything and purge celebrity and wealth, talented people vote with their feet and migrate to places where they can get rich and famous and say what they think. Those that remain settle for the safety of government-approved groupthink. As a result of this systemic dampening of creativity, the economy stagnates in the long run.
Aspects of contemporary AI theory align with the intuitions of Karl Popper as expressed in The Open Society and Its Enemies. Driven mostly by reaction to the totalitarian horrors of fascism and communism in World War II, Popper intuited that social truth is best served by policy competition and piecemeal social engineering not policy monopoly. Contemporary AI, in the form of discussion of the exploration/exploitation tradeoff in reinforcement learning (a variant of ML), explains why.
Exploitation is a strategy whereby the AI takes a decision assumed to be optimal based on data observed to date. In essence, it is about trusting past data to be a reliable guide to the future, or at least today. Exploration, by contrast, is a strategy that consists of not taking the decision that seems to be optimal based on existing past data. The AI agent bets on the fact that observed data are not yet sufficient to correctly identify the best option. Obviously, exploitation works better in closed and well-understood systems, but exploration is a better bet in those that are open and poorly understood.
Even if decisions are made by the most generally intelligent AI possible, the optimal strategy for that AI is to subdivide tasks, duplicate itself, and specialize for local conditions. In other words, a swarm of individuals each making their own choices can learn from the best of what its population tries. If all individuals are constrained, then the ability of the swarm to learn and change is crippled. There are exceptions, particularly where the cost of an individual failing is so high it is comparable to the whole population failing (for instance, letting more people have access to a button that ends the world is worse than letting fewer people have access to this button). But, generally speaking, more distributed control consistently beats more centralised control. By employing many different, often contradictory policies at once, we constantly explore as we exploit. Applying this recent technical insight retrospectively to history, it explains the sustained stagnation of Marxist economies.
Presently, no functioning state has either completely central or distributed control. We are all somewhere in between. In the mid-19th century, when The Communist Manifesto was published, there was hardly any spending on social services. Income tax was three percent in the UK, there was no such thing as company tax, and the welfare state did not exist. What existed was the parish and the poorhouse. In the days of Gladstone and Disraeli, with property-based suffrage and a budget than went mostly on the Army, the Navy, and servicing debt incurred during the Crimean War, one could plausibly claim, as Marx and Engels did, that “the executive of the modern state was nothing but a committee for managing the common affairs of the bourgeoisie.” In the 19th century, spending by the UK government was less than a 10th of GDP. Today it is a third. Half the UK budget, one-sixth of GDP, goes on health, education, and welfare.
A degree of central planning is desirable to provide infrastructure, to support basic research, and to ensure that everyone has access to education and health services. Regulation is required to enforce contracts, to facilitate cooperation, to provide minimum standards for products and services, and to enforce rules on safety, pollution, and so on. However, as an overall policy, maximising individual autonomy within reason, erring towards computational efficiency and distributed control, will yield dramatically better outcomes than central control by the AI of a one-party state.
Central planning ignores what is arguably the greatest advantage of distributed control and local adaptation: error correction. It also ignores the fact that “fairness” is notoriously hard to define in AI terms, assuming resources are to be fairly allocated. A central planner might select what is best for an average human, but what is best is often far from obvious. Humans are quite dissimilar from one another. We share goals only in the most general sense (for example, we seek to avoid pain, find food, take shelter, and so forth). We rarely agree on what we want to do today or any other day, and our beliefs about how to achieve things are often inconsistent.
The best possible central planner, mathematically, is a pareto-optimal super-intelligence. This is a software agent that learns faster to predict more accurately than any other agent, on average across all possible tasks. This is the theoretical upper limit of intelligence (allowing for debate over the exact definition of intelligence itself). However, even this theoretical perfection will always be out-performed by those with a more specifically relevant inductive bias toward a given task (those who are less intelligent in general, but more suited to the task at hand). In other words, even the most intelligent being possible would make mistakes when compared with the possibilities presented by distributed control, localised adaptation, and selective evolution. The same goes not just for correcting mistakes, but for improving our lot in life. Every beneficial innovation in history was an instance of an individual breaking ranks to correct a perceived flaw in the norm, to adapt to the specific situation at hand. Innovation requires disobedience. To centralise control is to encourage stagnation.
The problem with state-run monopolies is that they are inherently inefficient because they lack the error correction provided by competition. Markets provide error correction in the form of people deciding products suck and buying elsewhere. In the realm of ideas, error correction occurs when people say a party’s policy does not work, but this option is removed when free speech is curtailed. In China, those who criticise government policy (or government officials) disappear and get silenced. Only a lucky few like Peng Shuai have global profiles high enough to get noticed. Notwithstanding their claims about “participatory” democracy, in the one-party state, dissidents and innovators are purged in darkness.
The history of the communist world is replete with economic disaster. Millions died as a result of famines caused by Stalin and Mao. Marxist doctrine underlies the economic underperformance of China compared to Taiwan. AI cannot save Marxism, but it can be used by Marxists to serve their agendas of surveillance and social control. AI can be used to bring about the death of democracy and enable the rule of a digital Big Brother.
Forced to choose between AI oligarchs who make fortunes selling ads, regulated by elected governments that the people can replace, or a society ruled by AI platforms staffed with political officers who repress all criticism of the party line, the former is preferable.