Health
Who Will Save Us From Racist AI?
The researchers analyzed multiple databases and the findings were striking—the ML models were able to predict self-reported race (classified as Asian, black, and white) with astonishing precision.
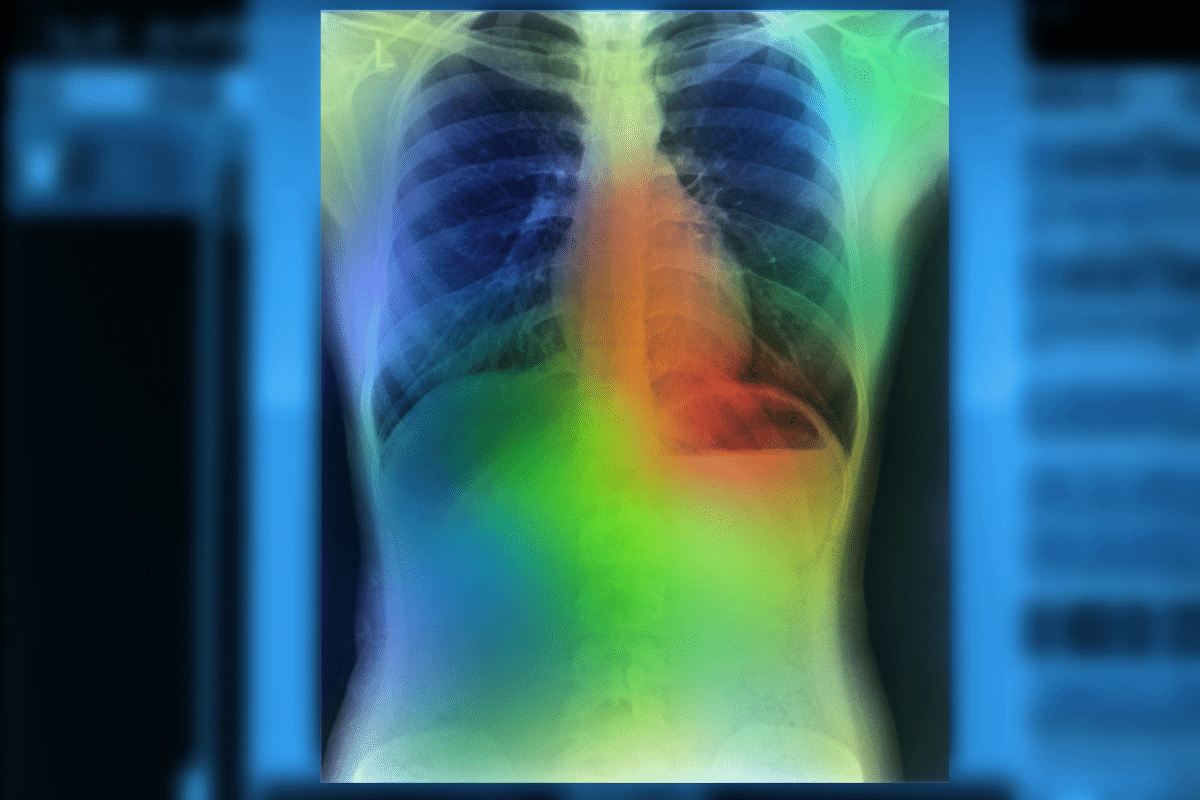
In recent years, a wealth of literature has emerged exploring how AI and machine learning (ML) can improve diagnostic precision in medicine. Combined with deep learning (a subset of ML), this research has the potential, inter alia, to advance cancer detection, streamline treatment algorithms, and enhance our ability to predict the risk of disease development. In brief, ML is the process by which AI can be trained to mimic the way humans learn, thereby improving its own accuracy over time.
As with any professional paradigm shift, controversy and spirited debates on ethics abound. Topics have included physician concerns that expert clinical decision-making may be forfeited to a computer algorithm with limited interpretability, the problem of ML systems often “overfitting” data (when an algorithm starts to measure sheer randomness rather than observable characteristics),1 and the integration of bias into any given ML program.2 The discussion of how medical bias relates to racial disparities in medicine is of particular concern in the modern era. However, a recent study regarding diagnostic imaging offers a reminder that this topic remains fraught with taboos and confusion.
The new preprint is entitled “Reading Race: AI Recognizes Patient’s Racial Identity in Medical Images,” and it details the use of ML to identify a patient’s self-reported race from routine radiographic studies (namely chest x-rays, computed tomography, and mammograms). The researchers analyzed multiple databases and the findings were striking—the ML models were able to predict self-reported race (classified as Asian, black, and white) with astonishing precision. This held true even when the researchers tried to account for other factors like breast and bone density or body mass index. The specificity with which the algorithm predicted race cannot be understated—each database revealed that findings never fell below 80 percent diagnostic accuracy, and many of the analyses found that measurements were accurate more than 90 percent of the time. These measurements held for different image resolutions and even when filters were applied to images by the researchers. The trained human eye, meanwhile, can detect race from such images at a rate no better than guesswork.
All told, it appears to be an exceptional piece of research and the authors were clearly alive to the ethical implications of the project. Unfortunately, discussions online have obscured at least as much as they have illuminated. Although there is no technical lead author for the paper, a member of the team named Dr. Luke Oakden-Rayner published a commentary on their findings in a thorough blog post entitled “AI Has the Worst Superpower…Medical Racism.” While the ethical concerns are well-articulated throughout Oakden-Ranyer’s post, his arguments sometimes lapse into self-contradiction, and the positive implications of his team’s findings are left unexamined.
Oakden-Rayner’s argument runs as follows:
- Medicine is biased against marginalized groups and in favor of white males.
- These biases cause medical disparities.
Therefore: - Racial bias in ML models will exacerbate those disparities.
He hypothesizes that the ML models are “primed to learn these features due to their inductive biases,” and refers to another preprint which found different true and false positive rates between racial groups in chest x-ray studies using the same ML models. Clearly, the model is learning to detect something the human eye cannot discern, and if false positive rates differ between racial groups, there is a potential for harm.
The potential for harm was the focus of an article in Wired, and preoccupied many of those who participated in the subsequent Twitter discussion about the blog, the paper, and its findings. A fellow researcher on the study from Emory University told the Wired journalist that the ability to identify race could lead to “inappropriate associations.” Another co-author remarked, “We have to educate people about this problem and research what we can do to mitigate it.” The authors seemed to share Oakden-Rayner’s general concern that their findings, ethically speaking, only pointed in the wrong direction. An additional study was referenced by the Wired journalist to emphasize racial disparities in the diagnostic accuracy of ML algorithms trained on chest x-rays.3
It is certainly important to consider how the use of such a model could affect care between population groups if false positive rates do differ. However, regardless of such concerns, some of the claims Oakden-Rayner made in his blog post and associated Twitter thread are needlessly confusing. Specifically, his statement that “the model has learned something wrong” and “the fact models learn features of racial identity is bad” lack meaning and validity unless one adheres to the orthodoxy that race is simply a social construct lacking any biological correlates.
This belief is exemplified in many of the supportive comments he received on Twitter. “I just want to note,” remarked a Stanford dermatologist, “that there are a lot of people in medicine, unfortunately, who still think race is biological rather than a social construct, and this paper shows that none of the biological attributes are predictive of race.” She re-emphasized this point by adding a screenshot from the preprint:
However, such defensive assertions puzzled other commenters who wanted to know why a model’s ability to identify a patient’s race is necessarily sinister in the first place.
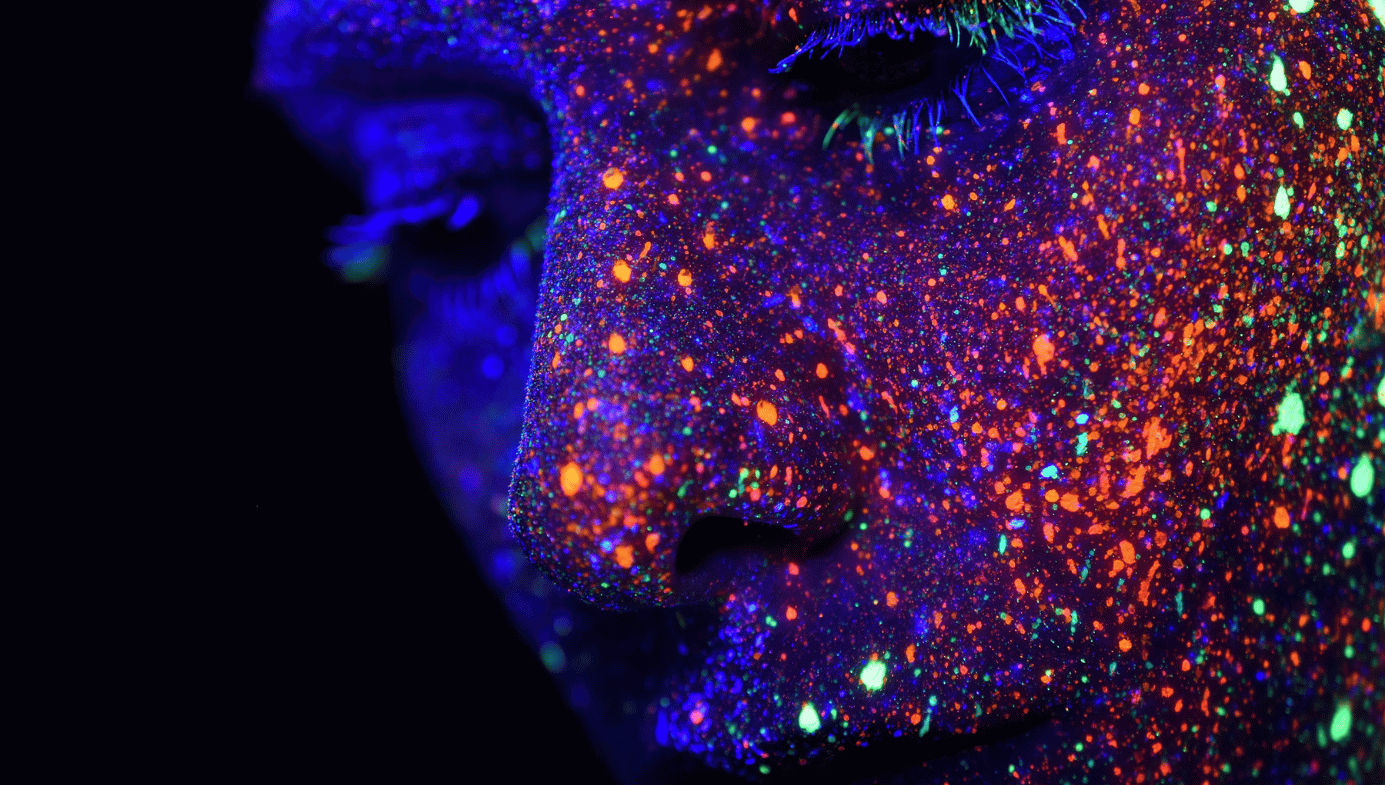
A review of the relevant literature reveals that, notwithstanding significant areas of overlap, biological correlates do differ between racial categories, and this is the rule not the exception.4 This has serious implications for treatment decisions, because adverse drug events can vary among population groups,5 as can disease rates. The most familiar example of the latter phenomenon is the sickle cell anemia trait, which is predominantly found in those categorized as “black” or “African American.” Average genetic differences between racial groups may also partially account for the higher incidence of aggressive prostate cancer in black men. Highly efficient and accurate ML algorithms will no doubt eventually pick up on these differences in a variety of circumstances, and it would therefore be unsurprising to learn that an AI was using average and subtle racial differences as a heuristic. The fact that the researchers tried to correct for differences like bone density at all suggests they are probably aware of this.
Many intellectually honest scientists already admit that race can be a useful proxy for some medical decision-making. If AI is prevented from accounting for this proxy, it could potentially produce more unintended harm than intended good. A recent medical controversy involving the African American adjustment for kidney function illustrates this point. One of the methods used to test a patient’s kidney function measures glomerular filtration rate (GFR). However, several studies have found that blacks have higher baseline GFRs than whites, so the test has to adjust for this factor depending upon the race of the patient. Graduate student activism led to several institutions removing the racial adjustment or replacing it with a different lab test, ostensibly in the name of addressing “systemic racism.”
Professionals justified this change with the same claim that race is simply a social construct. Nevertheless, it is not at all clear why simply labeling something a social construct automatically disqualifies it from medical algorithms—particularly given healthcare’s unending fixation on social determinants of health. This development was particularly distressing because a study published around the same time found that eliminating the racial adjustment resulted in less accurate estimates of kidney function in African American patients, with potentially harmful downstream consequences.6
Incorporating race in medical algorithms is not limited to kidney function estimations. In my own specialty, we often use a scoring system based on the Multi-Ethnic Study of Atherosclerosis to predict a patient’s 10-year risk of developing a type of heart disease to guide treatment decisions.7 Regardless of which organ system is evaluated, I would be willing to bet many patients would prefer not to have the racial/ethnic aspect of their testing excluded if it meant a less accurate risk prediction.
With this in mind, Oakden-Rayner’s historical account of medical trials’ bias towards white males arguably contradicts his expressed fears about AI racial recognition. If his claims of bias and exclusion against underrepresented groups are to be taken seriously, improving the accuracy of racial identification offers an opportunity for a massive and positive historical correction. It seems unlikely that data collected through imaging studies would be significantly more biased than other collection methods, and it may allow for diagnoses to be adjusted to produce uniform accuracy between groups.
Oakden-Rayner has stated that he doesn’t know how to change this algorithm to exclude race without making the ML model less clinically useful (a fascinating finding in its own right), but there remains an obvious concern about the integration of bias into any model. However justified this concern may be, the fervor around mitigating disparities is confounded with the refusal to acknowledge any average difference between racial groups. This is an unsustainable contradiction, and such moral panics waste valuable time creating alarmism around otherwise interesting research.
A finding such as this could be overturned with further study (for instance, it is not yet clear if this model works as well with Magnetic Resonance Imaging), or it could turn out that the algorithm is measuring an unknown proxy beyond race to make a distinction that only appears to be an identification of race. But until we know more and can assess the positive utility of such results, it is unnecessary to label potentially important outcomes like these as “wrong,” a priori. It is worth pointing out that not all the authors of the paper seem to have shared Oakden-Rayner’s concerns. As he stated in the introduction to his blog post:
One thing we noticed when we were working on this research was that there was a clear divide in our team. The more clinical and safety/bias related researchers were shocked, confused, and frankly horrified by the results we were getting. Some of the computer scientists and the more junior researchers on the other hand were surprised by our reaction. They didn’t really understand why we were concerned.
Regardless of the outcome (the article has not yet been peer-reviewed), ML functions best with a diverse data-set to optimize decision-making.8 Rather than breed alarmism, findings such as these will hopefully spur researchers to include as many members of varying populations in their trials as possible. It was encouraging to see Oakden-Rayner conclude his blog post with this same sentiment: “We absolutely have to do more race-stratified testing in AI systems, and probably shouldn’t allow AI systems to be used outside of populations they have been tested in.” We should proceed with caution before integrating AI at this level, but an equal amount of effort should be made to avoid surrendering to irrational fears or moralizing problems we have yet to understand.
References
1 Ascent of machine learning in medicine. Nat Mater. 2019;18(5):407. doi:10.1038/s41563-019-0360-1
2 Yoon CH, Torrance R, Scheinerman N. Machine learning in medicine: should the pursuit of enhanced interpretability be abandoned? J Med Ethics.2021;0:1-5. doi: 10.1136/medethics-2020-107102
3 Seyyed-Kalantari L, Liu G, McDermott M, Chen IY, Ghassemi M. CheXclusion: Fairness gaps in deep chest X-ray classifiers. Pac Symp Biocomput. 2021;26:232-243.
4 Murray, C. Human Diversity: The Biology of Gender, Race, and Class. 2020. p189.
5 Brunham, L., Chan, S., Li, R. et al. Pharmacogenomic diversity in Singaporean populations and Europeans. Pharmacogenomics J 14, 555–563 (2014). https://doi.org/10.1038/tpj.2014.22
6 Levey AS, Tighiouart H, Titan SM, Inker LA. Estimation of Glomerular Filtration Rate With vs Without Including Patient Race. JAMA Intern Med. 2020;180(5):793-795. doi:10.1001/jamainternmed.2020.0045
7 McClelland RL, Jorgensen NW, Budoff M, et al. 10-Year Coronary Heart Disease Risk Prediction Using Coronary Artery Calcium and Traditional Risk Factors: Derivation in the MESA (Multi-Ethnic Study of Atherosclerosis) With Validation in the HNR (Heinz Nixdorf Recall) Study and the DHS (Dallas Heart Study). J Am Coll Cardiol. 2015;66(15):1643-1653. doi:10.1016/j.jacc.2015.08.035
8 Rajkomar A, Dean J, Kohane I. Machine Learning in Medicine. N Engl J Med. 2019;380(14):1347-1358. doi:10.1056/NEJMra1814259