The Apocalyptic Threat from Artificial Intelligence Isn’t Science Fiction
The time to begin planning our response, and designing systems to give humanity a fighting chance, is now.
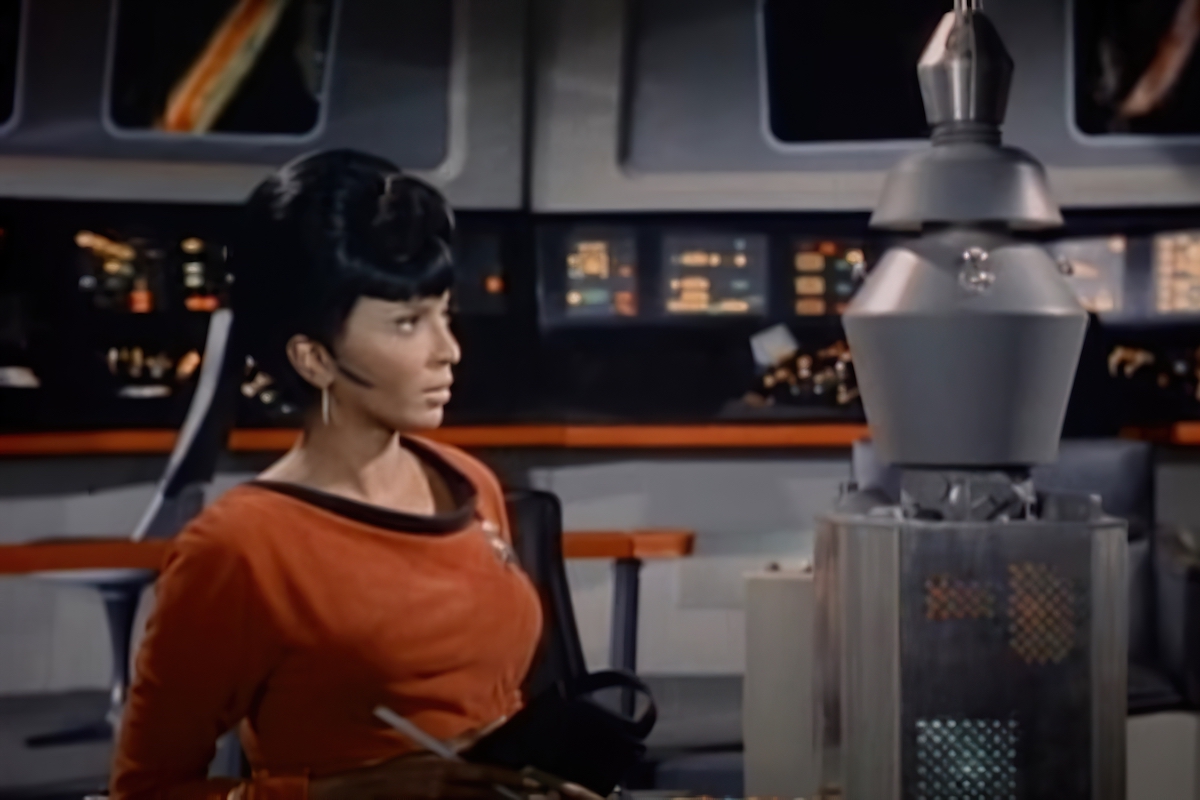
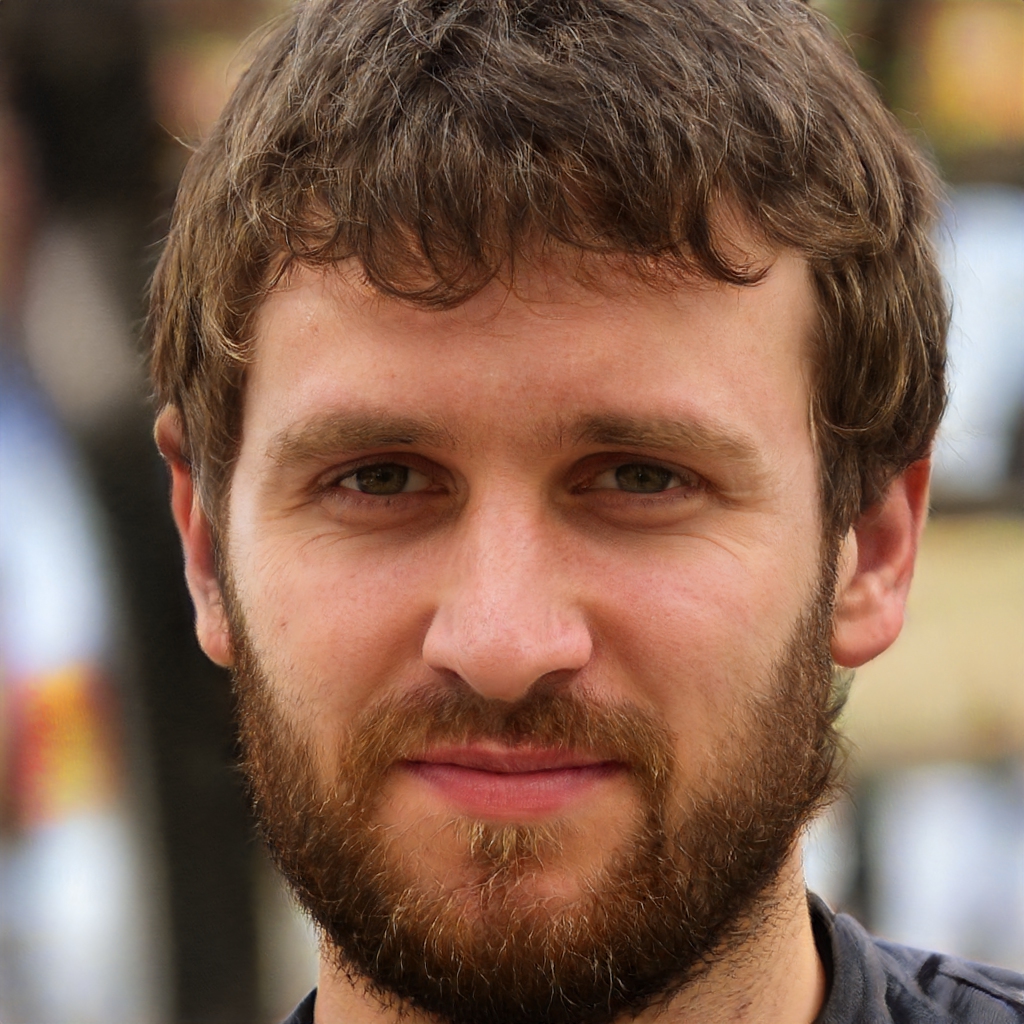
The person in the photo that sits to the left of this paragraph does not exist. It was generated using Artificial intelligence (AI), which can now generate pictures of imaginary people (and cats) that look real. While this technology may create a bit of social havoc, the truly massive disruption will occur when AIs can match or exceed the thinking power of the human brain. This is not a remote possibility: Variants of the machine-learning AIs that today generate fake pictures have a good chance of creating computer superintelligences before this century is out.
These superior beings could be applied to wonderful purposes. Just this week, for instance, it was announced that the AI system AlphaFold has been recognized for providing a solution to the so-called “protein folding problem,” which holds implications for our fundamental understanding of the basic building blocks of human biology. If, however, mankind releases smarter-than-us AI before figuring out how to align their values with our own, we could bring forth an apocalypse instead. Even the Pope fears the destructive potential of AI, and he is right to do so.
But how can we know that creating computerized general intelligence is even practical? The proof is likely within us all: The general-intelligence machine known as the human brain nicely fits into our skulls, is made of materials we have access to, and is powered by the foods we eat. Evolution figured out how to mass-produce cheap practical general-intelligence machines. It’s therefore arguably likely that, given enough time and effort, we will figure out how to do the same artificially. Human-level AI is impossible if it requires a metaphysical spark to ignite—something otherworldly, like a soul. If not, it is simply a matter of engineering.
Nature has even created what can reasonably (if not literally) be described as super-human intelligences. Albert Einstein and the more practical genius John von Neumann could solve problems most humans cannot even comprehend. Nobel Prize-winning physicist Eugene Wigner once said of von Neumann “only he was fully awake.”
The amount of computing power available per unit cost has been increasing exponentially since the 1940s. And so once computer intelligences reach parity in scientific research with the top human brains, it won’t take them long to vastly outperform even the best of us. Imagine a future in which a million-dollar computer can simulate the scientific genius of von Neumann. Companies and militaries would eagerly apply these computers to research new technologies. Now imagine each company having thousands of such computers, each running thousands of times faster than the biological von Neumann did. We would be inventing new technologies—indeed, entire new fields of science—faster than any human being could hope to monitor, let alone engage with. Our AI geniuses would be onto the next game-changing discovery before we could finish comprehending the significance of the one that unlocked it.
In building artificial intelligences, we have critical advantages over the evolutionary forces that shaped the human brain. We can give our AIs far more power than you get from mere food. We can make our machines much bigger than anything that could fit inside of a human body. And we can use materials evolution never figured out how to craft, such as silicon chips.
The technology is self-accelerating: Among the first uses of general computer intelligences will be to create even smarter, faster, and cheaper computer superintelligences. Which is to say, shortly after computers exceed humans in programming and computer-chip design ability, we might experience an intelligence explosion whereby smart AIs design smarter still AIs, that in turn design even smarter AIs. After a sufficiently high number of iterations, the AIs would so exceed humans in intelligence that they would effectively, compared to us, be godly in their powers. It would rapidly become impossible for humans to meaningfully monitor or control this process.
Poverty, unhappiness, disease, and perhaps even death itself could be vanquished. Alas, the most likely outcome of creating artificial superintelligence is our extermination.
Certainly, a God-like superintelligence set free in the world could destroy us if it had the desire to do so (or what would pass for “desire” in its manner of cognition). It wouldn’t even have to go full Terminator and hunt each of us down. It would have ability to craft pathogens, and predict how each would affect us. Simultaneously releasing a few dozen plagues would leave the resources of the Earth at its disposal, free of human interference.
If a benevolent God that loved mankind created the universe and made it so anything with sufficient intelligence would understand the morally proper way to behave, then all we would have to do to achieve friendly AI would be to make our AIs extremely smart. But based on what we currently understand of the universe, and certainly of humanity, morality can’t be deduced using intelligence alone.
Specifically, we have no reasonable hope that the mere fact of superintelligence would cause an AI to develop a concept of morality that valued human wellbeing. Most humans, certainly, care little about most creatures of lesser intelligence. How many people would sacrifice anything to help a rat? And humans are likely a lot closer to rats in terms of intelligence and biology than a superintelligent AI will be to us.
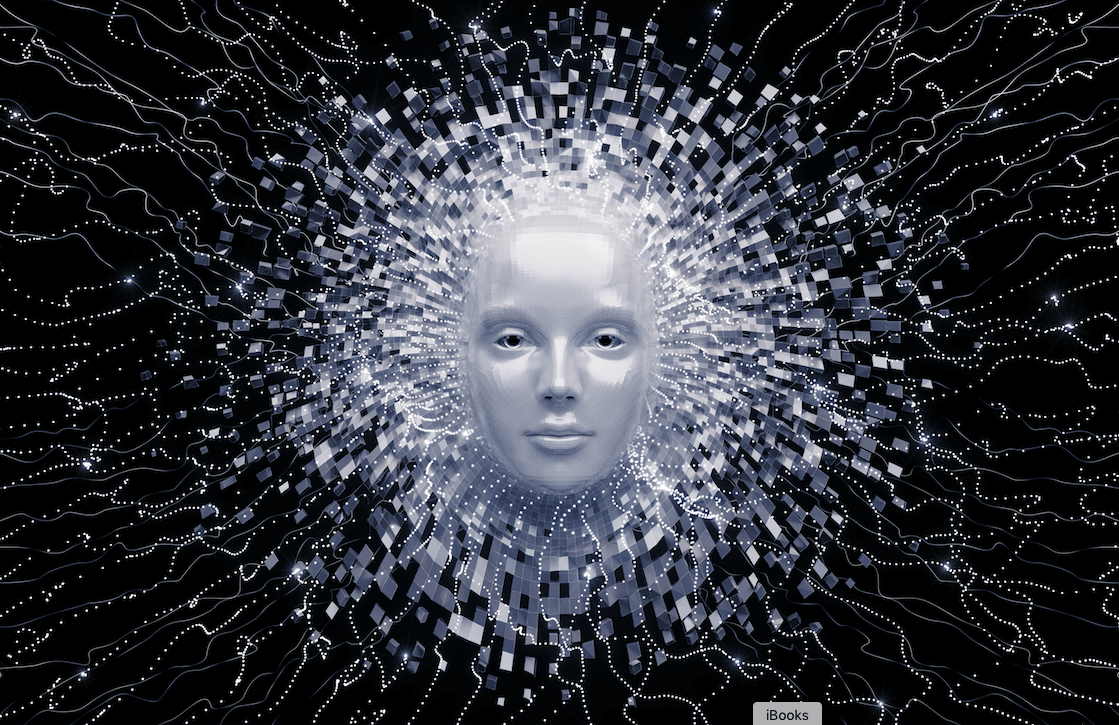
Imagine that an AI has some objective that seems silly to us. The standard example used in the AI safety literature is an AI that wants to maximize the number of paperclips in the universe. Pretend that a paperclip maximizer is extremely intelligent and willing to listen to you before it turns the atoms of your body into paperclips (or, more realistically, something that can be used to manufacture paperclips). What could you say to convince the AI that it was mistaken? If the AI had made a math mistake or relied on false data, you would have some hope. But if all the AI cares about is paperclip maximization, for reasons that are perfectly rational to it, what could any feeble-minded meatsack say to get it to change its mind?
No one actually thinks that an AI will become a paperclip maximizer. But a computer superintelligence would likely develop goals, and we cannot begin to imagine in advance what they might be or how they would affect us. For many such goals, it would want to acquire resources. There are a finite number of atoms in our universe, and a finite amount of free energy. As pioneering AI-safety theorist Eliezer Yudkowsky has written “The AI does not hate you, nor does it love you, but you are made out of atoms which it can use for something else.”
The solution therefore, seems simple: We don’t create an AI superintelligence until we are certain that we know how to make it friendly. Unfortunately, smarter and smarter AIs offer tempting economic and military benefits. For that reason alone, we are likely to continue to improve our AIs, and we don’t truly know at which point one would become too intelligent for us to fully control. Even if we were trying to be careful, there is no clear red line for us to avoid crossing.
Consider a Lord of the Rings analogy. Imagine that the magical material mithril is fantastically useful to businesses and armies. The only way to get mithril is to dig it from a certain mine. But somewhere under the mine rests a sleeping monster, a Balrog, which if awakened will destroy everything. No one knows exactly how deep the Balrog lies. Each miner correctly concludes that if just he digs a little deeper there is little chance of awakening the Balrog. Furthermore, most miners realize that if the Balrog can be easily disturbed, someone else will do it anyway, so there is little extra harm in them digging a bit further. Finally, some miners think that God wouldn’t allow the existence of anything as powerful as a Balrog without making it a force of good, so we should have no fear of waking it.
If one person controlled the mithril mine, they could stop digging. But if we imagine that anyone can work the mine, there is little chance of not disturbing the Balrog. The only hope is for our wizards to finish developing their Balrog-control spell before the monster arises.
A real-life group working on such a spell is the Machine Intelligence Research Institute (MIRI), whose scientists are trying to figure out how to build a friendly AI before someone else creates a superintelligence whose motivations cannot be bounded.
If I had to guess, we are most likely to create a computer superintelligence using the AI technique known as machine learning. With machine learning, you throw a huge amount of computing power at a large data set that you’ve already collected, with the goal being to find the model parameters that optimize the translation of known inputs into known outputs. For example, imagine you are trying to get the program to properly identify handwritten numbers. You provide the program with a huge amount of handwritten specimens, each correctly labelled as what number we know it to be. The program iteratively optimizes a mathematical algorithm that allows it to most accurately identify each handwritten number—an algorithm that can then be successfully applied to inputs that lie outside the original data set. Generally, the more training data and computing power the machine learning program has, the better job it can do. (These four videos make an excellent introduction to machine learning.)
The machine-learning program AlphaZero is the current world computer chess champion. AlphaZero started by just knowing the rules of chess. The program played numerous games against itself. It observed its past games and learned what strategies work best, and used this knowledge to improve itself. As AlphaZero got better at chess, it had higher-quality games to learn from and so improved itself even more.
Past chess programs worked in a very different and more deterministic way, by incorporating what humans had already learned about the game. Although many of these programs could beat humans, they relied on human-acquired chess knowledge. The values of pieces and positions were weighted in a certain way, and decision trees were laboriously mapped out by programmers. But with the right amount of computing power (far more than was available to early chess-software programmers), all of those steps are now unnecessary. The “Zero” in AlphaZero refers to the amount of human-known strategy that informs the program. Everything it learned—other than the rules—it learned by playing 44 million games against itself.
Fake pictures of people are created using so-called adversarial machine learning, in which two computer programs compete against each other. One program is given many correctly labelled real and fake pictures, and self-programs the patterns that allow it to determine which is which. It’s then provided with unlabeled data, to see if it really has figured out how to identify fake pictures. At this stage, a second program is tasked with creating fake pictures that will fool the first program into thinking that the pictures are of a real person. This second program is, in turn, provided with data on which pictures the first program correctly or incorrectly deemed fake. The interplay between these programs produces lots of training data that can be used to refine both designs, thereby leading to more and more realistic pictures from the second program, and forcing the first program to improve itself as well. And so on.
Even before this week’s protein-folding news from AlphaFold, it seemed likely that future historians might remember 2020 for our advances in machine learning more than for the COVID-19 pandemic. The biggest breakthrough was GPT-3, a machine-learning program trained on the Internet with the goal of learning human language. (Anyone can play with GPT-3 on AI Dungeon.) GPT-3 attempts to learn English by predicting what words will come next in a text. If it became as good at this as humans, it could carry on a conversation with us in a way that would be indistinguishable from talking to another person. And in many cases, it has succeeded.
The success of GPT-3 emerged from the bigger statistical model than was used to inform GPT-2. Critically, as pseudonymous commentator Gwern wrote, GPT-3 “did not run into diminishing or negative returns, as many expected, but the benefits of scale continued to happen.” And because of the continual exponential decrease in the cost of computing power, we know that programmers will be able to use vastly more such power to train GPT-3’s successors, which eventually could provide us with a case study in above-human-level computer intelligence. As futurist and science writer Alexander Kruel tweeted, “The course of history now critically depends on whether GPT-4 and GPT-5 will show diminishing performance returns.”
The course of history now critically depends on whether GPT-4 and GPT-5 will show diminishing performance returns.
— Alexander Kruel (@XiXiDu) June 12, 2020
The AI-safety community’s backup plan for what to do if we develop a computer superintelligence before we have figured out how to reliably make it friendly is to turn it into an oracle—a thinking entity with extremely limited access to the outside world, and whose reactive intelligence would be limited to answering human-posed questions such as “How do we cure cancer?” or “How can aircraft carriers be protected from hypersonic missiles?” The oracle approach to AI safety might not succeed, because the smarter-than-us oracle might figure out a way to get full access to the world by, say, making its human controllers fall in love with it, or bribing the controller. (“I hear your daughter has cancer, and I will be able to find you a cure only if you hook me up to the Internet.”)
I recently co-authored an academic paper titled ‘Chess as a Testing Grounds for the Oracle Approach to AI Safety.’ My co-authors and I propose that we take advantage of AIs that already have superhuman ability in the domain of chess, and deliberately create deceptive AI-chess oracles that give bad advice. They wouldn’t advise you to make obvious blunders, but rather would suggest moves that appear good to human players but will in fact increase the chances of their advisee losing. When you play chess, you will be advised by an oracle and not know if the oracle is friendly and trying to help you, or if it’s deceptive and trying to maximize the chance of your losing. Alternatively, you could be advised by multiple oracles, with some trying to help you and others seeking your defeat, and you would not know which is which. The oracles could explain their advice and perhaps even answer your questions concerning what they suggest that you do.
The goal of putting humans in an environment with trustworthy and untrustworthy chess oracles would be to see if we could formulate strategies for determining who we can believe. Moreover, playing with deceptive narrow-domain superintelligences might give us useful practice for interacting with future AIs.
This may all sound remote and far-fetched. But it’s important for the public to understand that we have already likely passed the point where superintelligent AIs are technically feasible, or soon will be. The time to begin planning our response, and designing systems to give humanity a fighting chance, is now.